Life appeared on earth approximately 4 billion years ago in the form of unicellular organisms known as microbes. Microbes are microscopic organisms including bacteria, viruses, fungi, archaea, and protists. Although microbes only received a brief mention in Darwin’s writings, they now offer strong testimony and evidence for the theory of evolution. Currently, most of the biodiversity on Earth is microbial and several genes, molecular machinery and metabolic pathways found in all living organisms evolved initially in microbes.
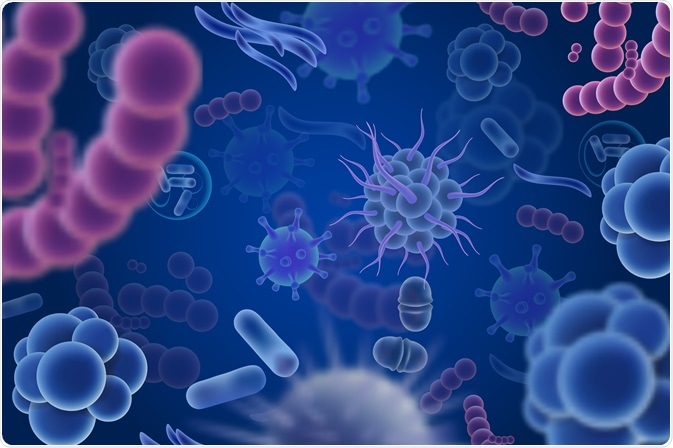
Image Credit: Siberian Art/Shutterstock.com
Although past efforts have been dedicated to studying intelligent processes in humans, other mammals, and birds, the topic the microbial intelligence has recently been currently gaining traction. Analysis of microbial models and comparative genomics studies confirm that microbes have evolved diverse means of memory, learning, and processing information; all of which are classified as ‘intelligent behavior’.
The most studied manifestations of intelligence in the microbial world include decision-making, problem-solving, associative learning, and quorum sensing. A better understanding of microbial intelligence can be utilized to solve human problems.
Decision making:
Microbes are able to monitor their environment, process information, and intelligently make a decision. These decisions can be made through various mechanisms and networks such as gene-expression regulation, signaling pathways, transport, metabolism, etc. There are ongoing studies involved in constructing genome-wide protein interaction networks to gain a better understanding of the molecules and interconnections required for microbes to make decisions.
The most well-studied example of microbes decision-making capabilities is the chemotaxis of E. coli. These microbes decide by monitoring their environment through plasma membrane receptors. If these receptors bind to certain ligands, a signaling pathway involving phosphorylation and methylation is induced within the cells. In this example, it is the level of phosphorylated CheY, a downstream protein of the signaling pathway, that ultimately decides which of two movements the E. coli cells undertake.
In the presence of an attractor ligand-receptor interaction, CheY is phosphorylated and binds to the flagellar motor. This causes the motor to rotate anti-clockwise, thus inducing a straight-swimming motion of E. coli cells. In contrast, in the absence of an attractor ligand-receptor interaction, CheY remains unphosphorylated and cannot bind to the flagellar motor. This causes the motor to rotate clockwise, thus inducing a tumbling motion of E. coli cells.
By utilizing this mechanism, E. coli can process information through their surroundings to decide whether to move towards or away from certain stimuli, ultimately increasing their chances of survival. For instance, this method allows E. coli cells to decide to move towards nutrients and away from toxic compounds.
You are your microbes - Jessica Green and Karen Guillemin
Problem solving
Using knowledge to solve new problems is an essential feature required to make an intelligent system. It is generally accepted that organisms with greater intelligence can solve more complex problems. Certain microbial systems have displayed problem-solving capabilities that can be utilized for survival. In some cases, these abilities can match or even surpass those displayed by humans.
An example of a microbial system that shows primitive intelligence through problem-solving is the slime mold Physarum polycephalum – a large amoeba-like cell consisting of pseudopodia. Studies have shown that these microbes are able to use their pseudopodia structures to connect food sources in different locations. More impressively, Physarum polycephalum can find the minimum-length pathway between the two food sources to obtain nutrients with greater efficiency.
Associative learning
Associative learning is a fundamental component of conditioning that involves learning associations between two stimuli or events. This process contributes to intelligence as it requires both learning and working memory. Multiple studies and models have confirmed that despite the lack of a nervous system, microbes can learn associations both individually and as a community.
The pathogenic bacteria P. aeruginosa is a well-established example of associative learning displayed in the microbial world. During surgical injury, ischemia or inflammation human tissues release several compounds into both the lumen and intestinal tissues. These compounds include interferon, opioids, and metabolites. Moreover, as the patient’s condition deteriorates, extracellular levels of phosphorus rapidly decrease.
P aeruginosa can detect these changes and has learned to associate them with patient vulnerability. This causes the bacteria to activate a lethal phenotype ultimately causing sepsis syndrome in the patient. Learning this association has caused P. aeruginosa to be the leading cause of infection-related mortality in hospitalized patients.
Quorum sensing
Bacterial cells can communicate and alter group behavior with one another by population density through quorum sensing, another manifestation of intelligence. This is a form of self-awareness that allows entire bacterial networks to recognize and adjust to particular environments collectively.
Quorum sensing involves the release of signal molecules known as auto-inducers which trigger transcriptional responses in target cells through receptor binding. This transcriptional response induces changes in the expression of various phenotypes and behaviors in the cells.
Quorum sensing was first discovered in Vibrio, a genus of gram-negative bacteria. In this instance when the bacterial cells are free-living, the concentration of auto-inducers is relatively low causing no change in phenotype. In contrast, when the population of bacterial cells exceeds the threshold, auto-inducer concentration rapidly increases and induces the transcription and expression of luciferase, thus leading to the bioluminescence of the cell population.
The benefits of understanding microbial intelligence
Understanding the mechanisms that underly microbial intelligence is an ongoing field of research. Scientists are invested in utilizing this knowledge to modify existing microbial networks or potentially create new ones to use to our advantage.
For example, extensive research is being dedicated to continuing to understand bacterial population dynamics including cell division, quorum sensing, bacterial secretion systems, and metabolite-sensing networks. Such knowledge can be used to design and develop novel antibacterial drug therapies to treat patients, especially since the efficacy of current treatments has declined over time due to antibiotic resistance in microbial populations.
Other interests include designing and improving biodegradation processes of microbes to use in wastewater treatment; as well as manipulating microbial metabolism for chemical production in biomedicine and food industries. Continuing to gain a better understanding of microbial dynamics and intelligence will allow for these ideas to become a reality in the near future.
References:
- Moxon, R. (2010). Darwin, Microbes, and Evolution by Natural Selection. Advances in Experimental Medicine and Biology. 697: 77-86.
- Nakagaki, T., Yamada, H. (2000). Maze-solving by an amoeboid organism. Nature. 407: 470-471.
- Pinto, D., Mascher, T. (2016). Bacterial “intelligence”: using comparative genomics to unravel the information processing capacity of microbes. Current Genetics. 62: 487-498.
- Steinert, M. (2014). Pathogen Intelligence. Frontiers in Cellular and Infection Microbiology. 4(8): 1-7.
- Westerhoff, H., Brooks, A., Simeonidis, E., Garcia, R., He, F., Boogerd, F., Jackson, V., Goncharuk, V., Kolodkin, A. (2014). Macromolecular networks and intelligence in microorganisms. Frontiers in Microbiology. 5(379): 1-17.
- Zaborin, A., Romanowski, K., Gerdes S., et al. (2009) Red death in Caenorhabditis elegans caused by Pseudomonas aeruginosa. Proceedings of the National Academy of Sciences of the United States of Amera. 106(15): 6327-6332.
Further Reading