The combination of digital pathology with immunohistochemistry is helping to resolve a major issue that has until now limited the accuracy and reliability of the immunohistochemical technique.
In a study conducted by scientists in Belgium, the issue of inter-batch variability of immunohistochemical staining was resolved by coupling it with digital pathology techniques.
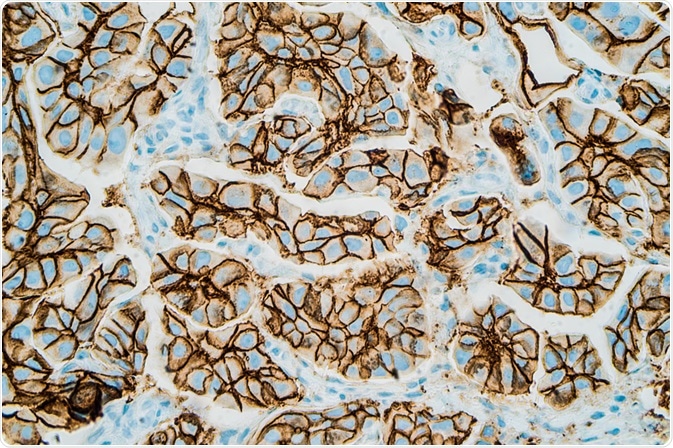
Image Credit: David. A. Litman/Shutterstock.com
The benefits of coupling digital pathology with immunohistochemistry
Histopathology relies on imaging tissue-based biomarkers to produce diagnoses, prognoses, and also to support its use in therapeutic purposes. In most of these cases, proteins are used as biomarkers.
The method of immunohistochemistry was developed to detect the presence of proteins that are related to a disease, illness, or particular biological trait, using stained antibodies that bind specifically to the antigens of interest (the proteins) that can then be visualized with imaging techniques.
For many years, pathological investigation has relied on the quantification of immunohistochemistry staining patterns to assess and monitor the disease, as well as drug efficacy.
Digital pathology is the discipline of digitizing data collected from imaging whole slide specimens and entering it into a digital database, where scientists around the world can access and share information.
Combining this approach with immunohistochemistry has become popular due to the benefits it brings, such as whole slide imaging as well as automated analysis and data sharing. These aspects reduce the impact of human subjectivity and enhance the accuracy and speed of immunohistochemical processes.
Also, a recent study has demonstrated that combining immunohistochemistry with digital pathology can overcome a significant problem facing the field: inter-batch variability.
Using digital pathology to solve inter-batch variability of immunohistochemical staining
Significant variations between batches of immunohistochemical-stained slides can arise even if laboratories control their workflow by observing strict protocols. This is known as the issue of inter-batch variability.
To ensure quantitative and reliable staining characterization across a complete series of slides, the variations between batches need to be minimized. Often, numerous batches are needed to process a large series of slides, and these become susceptible to the negative effects of inter-bath variation.
Scientists in Belgium recently published a study in the journal Scientific Reports in which they used digital pathology to solve inter-batch variability of immunohistochemical staining. The team recognized that variations are not always easy to visually identify, and therefore, a new method was required to distinguish these variations, preventing them from impacting on the data analysis.
To overcome this, the researchers established a technique of identifying variations and correcting them using a method of image normalization.
Normalization techniques have been heavily investigated for hematoxylin-eosin (H&E) staining, where results have shown that the specific tissue structures are visualized in a specific and consistent manner, for example, highlighting the nuclei in a blue/violet color and other cell organelles in other, specific colors. This allows shape information to facilitate stain identification.
However, the patterns of protein expression in immunohistochemical staining can show a great variation in factors such as location, intensity, and area, that can be impacted by the type of tissue that is analyzed and the protein that is of interest. Because of this, inter-batch variations that are not biologically relevant between immunohistochemical samples can be difficult to distinguish and to correct for.
To overcome this issue, the Belgium-based team designed a method that established staining references by using tissue microarray (TMA) slides that were sliced from the same block. Using this method, references were obtained for different immunohistochemical batches that targeted specific proteins.
The team extracted representative samples from each reference to determine the distinct characteristics of color and intensity.
The researchers then analyzed inter-batch variations to establish whether image normalization was needed, as well as to evaluate the efficacy of various image normalization methods that the team adapted for use in immunohistochemical staining.
Establishing the need for normalization
What is significant about what was achieved by the team at the Université Libre de Bruxelles, Belgium, is that they developed an experimental framework to objectively evaluate the need for normalization of images in immunohistochemistry, as well as to identify the most appropriate way to perform this normalization.
The team emphasized the need to improve quantitative evaluation and comparison, rather than just obtaining visual improvements in the process.
The methodology that was established with the help of tissue microarray (TMA) materials coupled with statistical analyses enabled the reliable and accurate evaluation of possible variations occurring in the color and intensity levels of samples. It also was able to efficiently analyze different methods of normalization and correct them.
The established methodology is likely to transform standard protocol in immunohistochemical staining, allowing for the extraction of quantitative features that characterize the staining patterns obtained by immunohistochemical staining. The study emphasized the benefit of combining digital pathology with immunohistochemistry.
Sources:
- Helps, S., Thornton, E., Kleinig, T., Manavis, J. and Vink, R., 2012. Automatic Nonsubjective Estimation of Antigen Content Visualized by Immunohistochemistry Using Color Deconvolution. Applied Immunohistochemistry & Molecular Morphology, 20(1), pp.82-90. https://www.ncbi.nlm.nih.gov/pubmed/22157059
- Van Eycke, Y., Allard, J., Salmon, I., Debeir, O. and Decaestecker, C., 2017. Image processing in digital pathology: an opportunity to solve inter-batch variability of immunohistochemical staining. Scientific Reports, 7(1). https://www.nature.com/articles/srep42964#citeas
- Williams, B., Jayewardene, D., and Treanor, D., 2019. Digital immunohistochemistry implementation, training, and validation: experience and technical notes from a large clinical laboratory. Journal of Clinical Pathology, 72(5), pp.373-378. https://jcp.bmj.com/content/72/5/373
Further Reading