The examples of AI and its applications in healthcare potentially offer affordable healthcare, efficient clinical trials, improved success rates, and a better quality of life. While many of us may be familiar with AI in the context of self-driving cars, Siri, or Alexa, we are beginning to slowly comprehend the full potential of AI’s clinical applications. AI’s steadily increasing impact in the healthcare industry can be demonstrated by analyzing the following five sectors of the industry.
1) AI Assistance in “Keeping Well”
One of AI’s biggest potential applications in healthcare, which promises to significantly impact the consumer directly, is its ability in aiding people to maintain their health. Growing significantly in the last ten years, the Internet of Medical Things (IoMT) application is on the rise. Healthy behavior in individuals is encouraged by healthcare apps. Mainstream companies such as Apple are beginning to make the tracking of cardiac health, emergency SOS, and fall detection significant products in their portfolio.
Consequently, it seems as though IoMT is here to stay. Allied Market Research has published a report suggesting that by 2021, the market for IoT healthcare will reach $136.8 billion worldwide, with a CAGR 12.5% between 2015 and 2021.
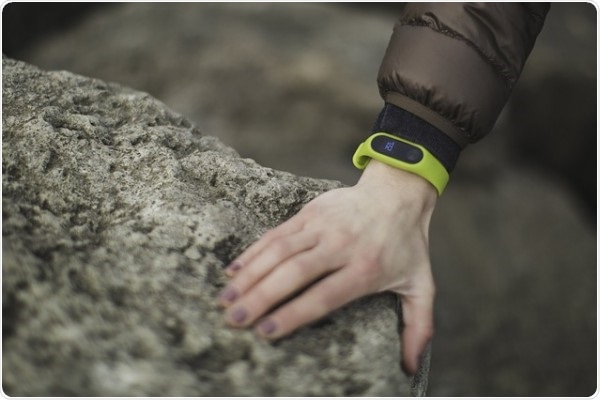
2) AI-Assisted Robotic Surgery
While it is still in its infancy, AI can improve surgical performance in terms of practice. In general, the outcome of surgeries – especially new or complex procedures – can vary depending on the surgeons’ skill. Case-to-case variations can be reduced by the use of AI, which can even help to increase even the best surgeons’ efficiency.
As an example, a three-dimensional magnification can be provided by robots controlled by AI, for performance and articulation with greater precision and miniaturization. Basic acts of precision cutting and stitching can be performed by AI enabled robots. Surgeons were seen using AI assisted robotics in 2017, in order to suture exceptionally narrow blood vessels – 0.03 to 0.08 millimeters across – at the Maastricht University Medical Center in the Netherlands.
The surgeon, of course, still retains control of the robotic suturing. There are a number of small, complex tasks throughout a surgical procedure which certainly require the nuanced skills of a surgeon. Before we are able to witness an AI utopia in which robots replace nurses and surgeons, we still have a long way to go. For now, however, they are fantastic assistants who can reduce the variability of outcomes.
3) Clinical Judgement or Diagnosis
The early detection of diseases such as cancer and retinopathies can and is already being improved by AI. By using AI to analyze and review radiology and mammogram images it is possible to increase the process’s speed as much as 30 fold, with a 99% accuracy. A study which described the successful use of AI algorithms in order to detect skin cancer against the diagnoses of 21 dermatologists was published by Stanford University in 2017. This year, a neural network was trained to detect over 50 types of eye disease by Google’s DeepMind technology. This was part of a collaborative study with the joint research of Moorfields Eye Hospital in London, UK, and worked via the analysis of 3D retinal scans. This study’s biggest advance upon the work of its predecessors is the greater ability to explain how the computer reached some of its interpretations. The trust and reliability of this application are reinforced by the overcoming of a previous black box of data interpretation and inference.
It is important to acknowledge and emphasize most of all the benefit of combining the powers of physicians with those of the AI algorithms. A competition took place at the International Symposium on Biomedical Imaging which competed for computational systems which were programmed to detect metastatic breast cancer from biopsy images against each other. The winning program in this competition made the diagnosis with a success rate of 92.5%, however, when combined with the opinions and expertise of human pathologists, that number increased to a success rate of 99.5%.
4) Precision Medicine
Precision medicine is one of the most valuable examples of the use of AI in healthcare. It is currently being touted as a paradigm-shifting healthcare practice. The enormous amount of data which is collected from many disruptive technological innovations, including cheap genome sequencing, advanced biotechnology, and health sensors patients use at home, is the bedrock of precision medicine.
The definition of precision medicine is the "tailoring of medical treatment to the individual characteristics of each patient." Medical practices are shifting quickly towards adopting a more personalized format for making decisions which were previously based on a few seemingly overlapping features among patients.
Precision medicine makes use of physicians' cognitive capabilities on a new scale as it depends on advanced supercomputing algorithms with deep learning. In the present climate in which it is simple to access genomic data, one of the challenges faced is persevering in order to identify genetic variants which increase the risk of disease. Collaborating with California based Scripps Research Institute, Intel has developed a deep learning algorithm which was able to detect 23 patients who had increased risk of cardiovascular disease. These patients were not identified by conventional statistic methods, and the algorithm detected them with an accuracy rate of 85%. With the ultimate aim of creating a "cognitive assistant” which has a range of clinical knowledge, analytical and reasoning capabilities, Google DeepMind and IBM Watson lead the globe in the mining of medical records.
5) Drug Discovery
Clinical trials in their current format encompass decades of research and cost billions of dollars. “Only five in 5,000 of the drugs that begin preclinical testing ever make it to human testing and just one of these five is ever approved for human usage,” according to the California Biomedical Research Association.
Pharmaceutical companies can be aided in the streamlining of drugs, as well as drug repurposing, by using AI in drug discovery. Sanofi, Genentech, Pfizer, and many other pharma giants are beginning to form partnerships with AI service providers – Exscientia’s Artificial Intelligence, GNS Healthcare, and IBM Watson respectively – in order to drive their oncology drug discovery programs.
Causes of various diseases which were previously unknown can be pinpointed by AI, which can then enable the testing of more compounds with a higher degree of accuracy and reproducibility. We would be able to do away with the traditional trial and error approach if we used AI for drug discovery, and consequently embrace a biology which is more patient-driven by using more data-derived predictive hypotheses.
In 2016, a drug development company called Atomwise used AI to analyze whether or not existing medicines could be redesigned to target the Ebola virus. The analysis was accomplished in a single day and led to two potential hits. Via conventional means, this process would have taken months or even years. Although in silico modeling techniques are becoming more important in modern research and development and drug research, they are still a long way from replacing the standard pharmaceutical-industry R&D productivity.
While the practices mentioned above constitute some of the “cooler” AI applications, working behind the scenes unnoticed is AI’s administrative workflow assistance, which has an estimated value of $18 billion.
AI has helped in the routine data collection jobs, recording, and long-term storage of doctors and nurses by mobilizing the automation of administrative jobs. AI may be able to give physicians more time to see patients regularly, by doing away with the repetitive parts of the caretaker’s job.
Ethical Concerns
The flip side of the flashy elements of AI in healthcare is issues concerning data privacy and its ethical usage. Some ethical issues concerning the use of AI include, but are not limited to, the following:
- If machine errors led to the mismanagement of care, who would be held accountable?
- Would pre-existing biases (for instance, over- or under-represented patient subgroups) inherent within the data used to train the AI, reinforce biases in diagnoses and analyses rather than eliminating them?
- Would patients be told about the extent to which AI was playing a role in their treatment?
- Would AI indulge in self-diagnosis and medication, rather than encouraging patients to seek advice from a medical practitioner?
- Could healthcare practitioners feel that AI potentially threatened their authority and autonomy? Would their medical practice be affected by this in turn?
Evidently, AI, as a newly developing technology, is a tightrope which requires careful treading. If the relevant ethical and data privacy criteria are kept in mind, and it is used responsibly, AI has the potential to make unprecedented transformations in how the healthcare industry works.
While we are still in the process of transitioning towards its use, medical professionals must be trained about the use of AI. AI is currently a buzzword which is frequently over-hyped, so it is important to avoid being bamboozled by realizing what does and does not actually help. AI may not be anywhere close to eliminating human involvement in the healthcare sector, however, jobs could surely start to favor practitioners who are educated about and accepting of AI in the sector.
Kolabtree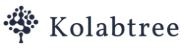
Kolabtree is the leading online marketplace for freelance scientists and researchers. Their global pool of 5,500+ freelance experts are typically PhD-qualified and are from institutions like MIT and Harvard University. Services offered include statistical analysis, scientific consulting, data analytics, scientific writing and literature search. Kolabtree has helped businesses around the world, including Volkswagen and Sage Publishing, access scientific expertise quickly and easily. The platform lets you choose a budget of your choice, and interact with freelancers before making a hire. It's free to post your requirement on the project and receive quotes from experts. Kolabtree’s freelancers have helped businesses develop a product, write a technical report, perform a data analysis, and more.
Kolabtree is a one-stop resource for entrepreneurs, businesses and research organizations to get quick help from experts at short notice.
Original piece written by Maya Raghunandan. Maya Raghunandan obtained her Ph.D in Biochemistry and Molecular Biology from the University of Minnesota, USA. Currently, she is a cancer biology scientist at Université Catholique de Louvain, Brussels, Belgium.
Sponsored Content Policy: News-Medical.net publishes articles and related content that may be derived from sources where we have existing commercial relationships, provided such content adds value to the core editorial ethos of News-Medical.Net which is to educate and inform site visitors interested in medical research, science, medical devices and treatments.