Dynamic light scattering (DLS), the primary biophysical technique for high-throughput screening of aggregation, is widely used by PIPPI.
In this interview with News-Medical and Life Sciences, Lorenzo Gentiluomo detailed the variety of DLS methods used to evaluate stability, including some novel applications. He also explains how the development of high-throughput technologies, such as DLS, has hugely increased the opportunity for finding patterns in data and the role of machine learning in this process.
Can you explain the role of light scattering in PIPPI’s research?
The PIPPI consortium is compiling a database of 18 representative proteins to characterize the critical attributes for successful formulation development. It will include the basic characterization of 24 formulations as a function of salt concentration and pH. In addition, there will be more in-depth molecular characterization of some of the formulations, where the structural properties of the protein will be related to solution behavior.
An important part of this work is the study of protein-protein interactions using dynamic light scattering (DLS) and static light scattering (SLS). We measured protein interactions using Wyatt’s DynaPro Plate Reader, which has temperature-controlled microwell plates.
The DynaPro Plate Reader can take both DLS and SLS measurements of the size, molecular weight, and interactions of proteins, providing a good understanding of the physical stability of a protein formulation. Size, molecular weight, polydispersity, viscosity, and interaction parameters can all be determined and followed by formulation conditions and temperature.
We measure protein-protein interaction using DLS by following the concentration dependence of the translational diffusion coefficient, kD. By simultaneously measuring SLS, we can also determine the strength of the pairwise intermolecular interaction, that is the second virial coefficient A2 or B22. This provides a good way of ranking formulations for colloidal stability.
There are two theories used to describe the pairwise protein-protein intermolecular interaction. I prefer using the proximity energy framework, i.e. the differences in potential energies which reflects the distance dependence. Increasing ionic strength shields the charge-charge interaction and should give a faster aggregation as it is easier for the molecules to interact with each other.
The diffusion interaction parameter kD has shown itself to be a key biophysical property for formulation; it is not only a measure of protein interaction. Recent measurements of kD for an antibody formulation revealed a relationship between kD and the apparent solubility, the thermal stability, the rheology and the electrostatic properties of the formulation.
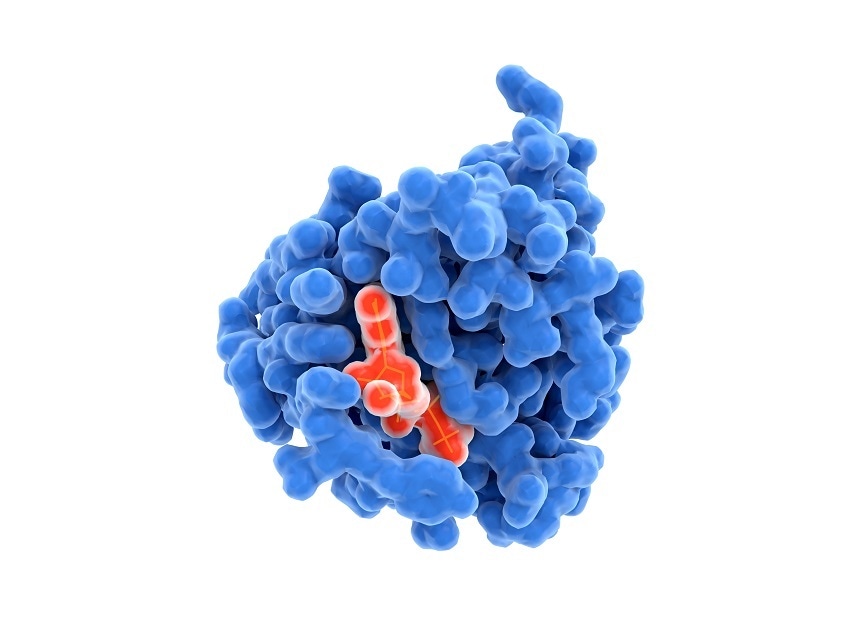
Image Credit:Shutterstock/JuanGaertner
Which properties of the proteins did you characterize?
We characterized the 24 formulations of 18 proteins as a function of pH and ionic strength, then applied a computational screen which calculated the 3D structure of the proteins in each of the 24 different formulations with relative molecular descriptors. From the screening, we gathered a series of outputs. Some are from the stress assay that was carried out by DLS and also by size-exclusion chromatography coupled with multi-angle light scattering (SEC-MALS).
An important parameter is the aggregation pattern when exposed to thermal stress, as this can give us a better understanding as needed for problem-solving during formulation development. To assess the thermostability of the proteins, aggregation was measured as a function of the pH and ionic strength. The same concentration of buffer was used but differing amounts of salt were added to change the ionic strength. The temperature at which aggregation occurs (Tagg) is measured by SLS. It should be noted that Tagg does not always correlate with structural stability, as aggregation can occur before the protein has started to unfold (as determined by intrinsic fluorescence).
The pattern of aggregation can tell you how the protein behaves. For example, if the aggregation occurs in two steps with a plateau in between, this indicates that the protein most probably has an equilibrium between the monomer and the complex—it is not only temperature dependent.
We also use light scattering to assess protein-protein interaction and how it changes with different ionic strengths. The shape of the kD curve is typically a function of the pH and is flattened by the addition of salt. When I see such a flattening, I know that the aggregation is led by hydrophobic or dipole interactions.
In some cases, the result from the size-exclusion chromatography and the result from the DLS from the native sample disagree. It is important to take both measurements into account to get a good understanding of a formulation.
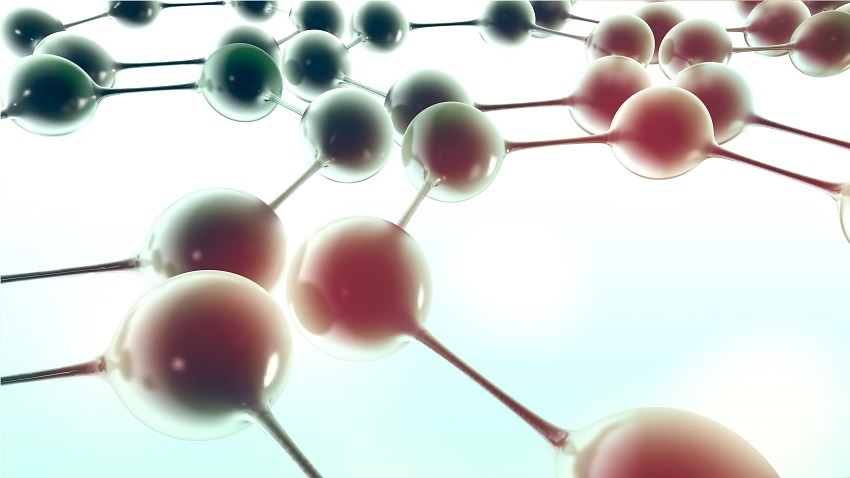
Image Credit:Shutterstock/SmirkDingo
How do you use such information to evaluate a formulation?
Once we have measured the various outputs, we can compare them with structural information using homology modelling. In this way, we can figure out why the surface is changing. If we see that kD is trending in a certain way for different antibodies, we can assess whether this is due to, say, a hydrophobic patch.
Our ultimate aim, though, is to use molecular descriptors to try and generate a global algorithm that can be used to predict outcomes for a particular protein. Our systematic approach should help to indicate which are the key biophysical property for protein formulation and the application of data mining techniques. We really believe that our database can enable a rapid and cost-sparing assessment of development potential.
During the screening, 75% of the total data points were collected using the DynaPro Plate Reader and SEC-MALS, where the latter consisted or an HPLC setup coupled to a Wyatt miniDAWN MALS instrument and Wyatt Optilab differential refractometer. So, you can see the contribution of light scattering to protein formulation development is quite significant.
What is the new approach you used to study protein stability?
We used the DynaPro Plate Reader to study the physical stability of a monoclonal antibody in a novel fashion.
First, the antibody was incubated in a denaturant, guanidinium hydrochloride, which allows us to follow the perturbation of the structure by intrinsic protein fluorescence using the isothermal chemical denaturation technique (ICD). We then applied a novel method, ‘dilution from denaturant (DFD)
By subsequently diluting the denaturing solution with buffer, we can assess the level of physical stability. If physical stability is low, the final solution will have larger aggregates or more aggregates than if it is high. When we compared DLS measurements with SEC-MALS analysis, we found that a higher hydrodynamic radius is positively correlated with more aggregates. Since dynamic light scattering is both very sensitive to aggregate detection, and (unlike SEC-MALS) a high-throughput technique, it provides the ideal method for evaluation in such a study.
Using two different buffers—histidine and citrate—but the same pH, the conformational stability as assessed by ICD was very similar. However, using DFD we were able to differentiate ultimate stability by DLS and hydrodynamic radius, which is related to colloidal stability. The value of this is that we just need one value, the hydrodynamic radius, to get the whole picture.
From our point of view, tracking aggregation using the DynaPro Plate Reader after dilution from different concentrations of a denaturant is a very promising approach to probe the physical stability of a protein in different formulation.
The DFD method is isothermal so we avoid all the problems arising from sample heating, for example, the shift in buffer pH that may occur. It requires only a very short measurement time and as such has a high potential for scale-down and automatization. Moreover, it can distinguish between overlapping ICD curves. This means that you can distinguish two formulations that have the same conformational stability by quantifying their colloidal stability with little extra effort.
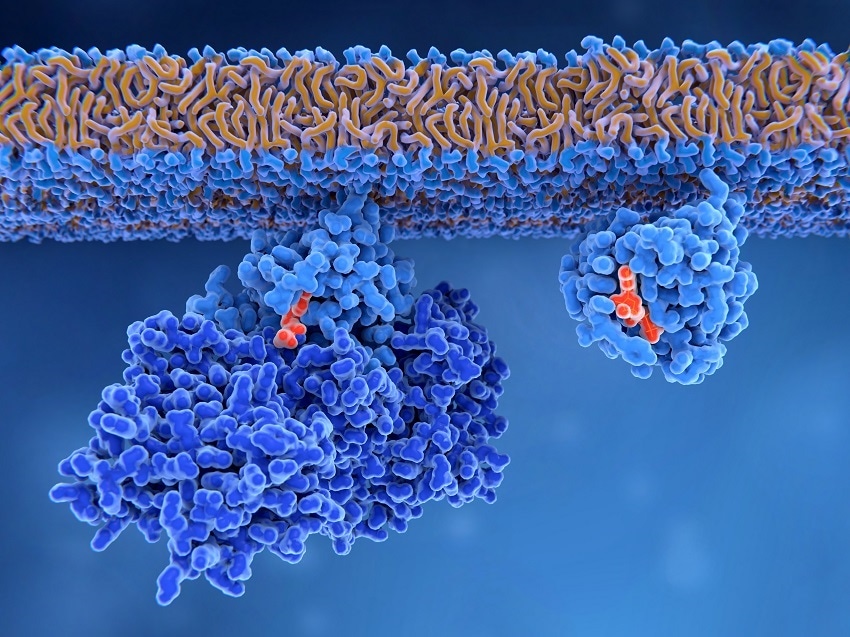
Image Credit:Shutterstock/ JuanGaertner
How does the machine learning algorithm fit in?
The machine learning algorithm is the application of an artificial neural network to protein formulation development. An artificial neural network has the capacity to deal with the highly neural linear problems that are often encountered during pharmaceutical development.
We developed an artificial neural-network algorithm to improve our understanding of protein solution behavior. We feed data into the algorithm and it predicts an outcome. For example, if we input information about the primary sequence and the formulation, we would get back the temperature of aggregation.
The artificial neural network thus represents a very interesting alternative to classical statistical methodologies. When applied to a highly non-linear data set it can be fairly successful and it is this kind of dataset that is most often encountered in pharmaceutical development.
It could be applied to optimize biologics based simply on amino acid composition. This would allow selection of protein structure with good predicted Tm, Tagg, kD, and in certain cases of monomer retention, even before they're expressed in cells.
Using various models, we have validated the algorithm and found that the prediction of Tagg is very accurate. It also accurately predicts whether kD is positive or negative (i.e., the proteins are self-repulsive or self-attractive, corresponding to colloidal stability or instability, respectively). The regression problem is very difficult to solve, so we focused on the sign of kD rather than deriving a quantitative value.
We were also interested in predicting the monomer retention or loss after stress. Predictions of monomer retention, i.e. the fraction of material that did not aggregate or fragment under stress, are also fairly accurate.
Lots of background noise meant it was difficult to distinguish between different formulations. We could, however, predict if one protein is more stable than another. Since this is predicted from the primary sequence, we can produce a relatively reliable estimate of the stability of candidate biologics without having to express and make direct measurements for every protein.
A major drawback of the neural network is the interpretability. The output from the neural network is not describing an actual algorithmic process, but an interpretation of the data entered into the algorithm. It does help us draw correlations though, which can give us further information about the process.
In summary, high-throughput light scattering is the perfect tool for screening and in-depth characterization of protein physical stability. Once completed the PIPPI database will facilitate the rapid assessment of a protein for suitability for development. Similarly, the machine learning algorithm will provide data-driven predictions to inform these decisions and enable cost-savings during the development of novel protein therapeutics.
Where can our readers go to find out more?
To find out more please visit;
www.wyatt.com/PlateReader
ww.wyatt.com/Biotherapeutics
About Lorenzo Gentiluomo
.jpg)
Lorenzo Gentiluomo is Master of Science and PIPPI consortium fellow for Wyatt Technology.
PIPPI, which stands for Protein-excipient Interactions and Protein-Protein Interactions, is a European consortium of experts from academia and industry that is working to address the challenges of protein-based drug formulations. It comprises a group of companies and universities in Europe and is led by the Technical University of Denmark.