Introduction
What is Real World Data?
Experimental Data vs. RWD
Difficulties with RWD
Benefits of RWD
Real-World Effectiveness
Drug Repurposing
Safety Evaluation
What Lies Ahead for RWD?
References
Healthcare is more of an art than a science since people are so different from each other. Today's gold standard of safety, potency, and efficacy regarding healthcare practices is the randomized controlled trial (RCT). Yet it is essential to remind ourselves that the best-designed trial yields statistical data, but human beings are not statistics.
The development of a new therapeutic is a long-drawn-out process and exorbitantly expensive in most cases. Drug approval takes years of evaluation via RCTs to ensure the proposed drug is safe and effective and better than existing drugs in either of these measures or cost-effectiveness.
The risk of complications or adverse effects from newly approved drugs is often rendered less apparent or missed altogether by using statistical measures from a clinical trial. One way to overcome this issue is to use real-world data (RWD).
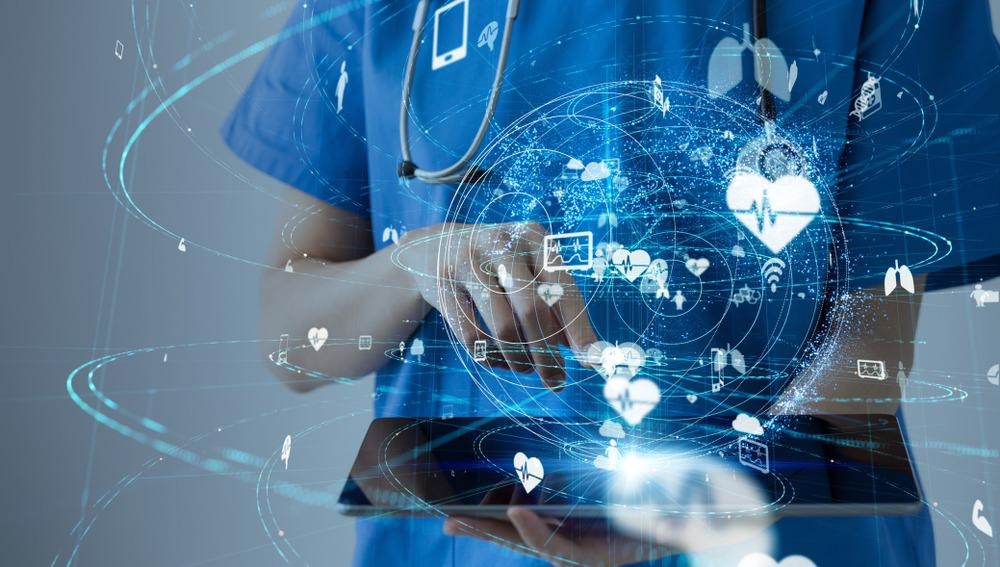
Image Credit: metamorworks/Shutterstock.com
What is Real World Data?
RWD includes all "observational data generated routinely during healthcare provision and exclude data generated experimentally," such as during a randomized clinical trial (RCT).
RWD is thus data linked to patients' health coming from sources other than RCTs, which can be processed to glean real-world evidence about the actual benefits and risks of a drug or product used in healthcare.
"Real-world evidence is the clinical evidence regarding the usage and potential benefits or risks of a medical product derived from analysis of RWD."
US Food and Drug Administration (FDA)
The sources of RWD include:
- Electronic health records (EHRs)
- Healthcare claims and bills
- Drug and disease registries
- Wearable sensors, mobile apps, and computer-based home patient data
Experimental Data vs. RWD
A real-world study is based on populations as they really occur in a clinical setting, with considerable heterogeneity, unlike the often optimized patient populations used in clinical trials. This includes people with multiple illnesses or risk factors, which may or may not overlap; varying family histories; and different degrees of prior healthcare access.
An RCT, conversely, includes a narrowly defined study population and may therefore not be readily extrapolated to other settings. The cost of such prospective randomized studies may be so high as to rule out sufficiently large numbers of participants, especially to detect less common outcomes.
More seriously, they do not provide a complete picture of the factors that may guide clinical decisions or help decide patient care. The follow-up period may be too short to identify the intervention's actual clinical or financial impact. Still, RCTs are widely accepted as having impressive internal validity, such as offering a high degree of confidence that the relationship between cause and effect is real and not artefactual.
Secondly, RWD comes from much larger populations than can be enrolled in clinical trials. And thirdly, it comes from the actual use of the intervention in less-than-ideal conditions, which is how the drug is most likely to be used. In this respect, RCTs lack external validity to varying extents; they are not fully generalizable to populations, more or less unlike the RCT study population, situation, or events.
Finally, using RWD may help understand some benefits or potential uses beyond those that were focused upon as outcomes in the RCT. RWD may allow for long-term follow-up of patients on medication compared to a typical RCT.
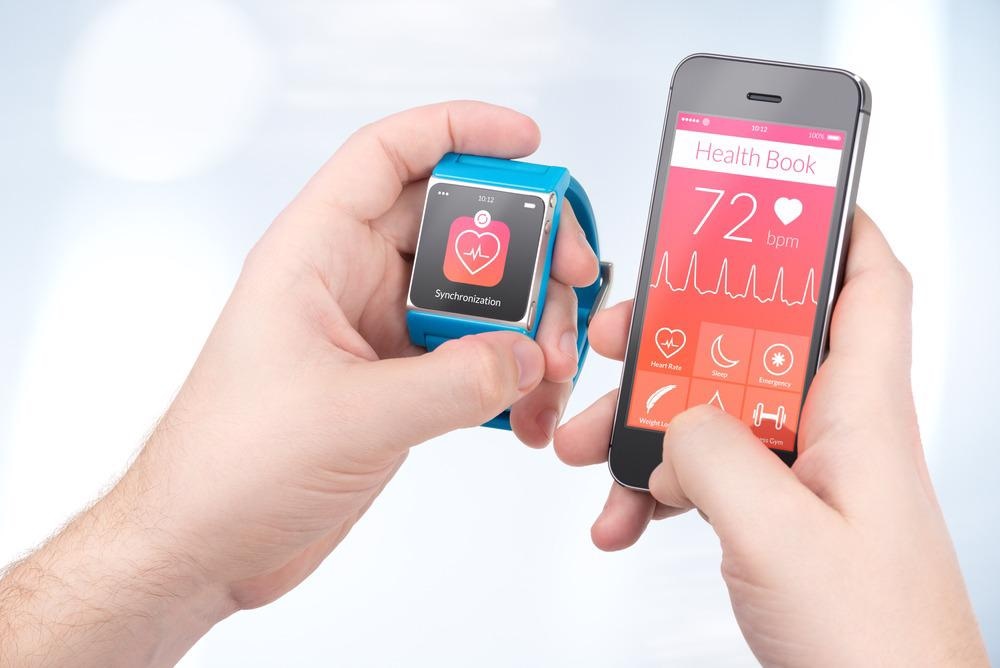
Image Credit: Alexey Boldin/Shutterstock.com
Difficulties with RWD
However, RWD sources are heterogeneous as to quality, coverage, and focus. They may range from EHRs to social media and mobile phone apps. There is a real danger of missing or mistaken data, and non-standardized formats make direct comparisons difficult. Observed associations may not be causal, as there are many possible confounding factors.
Recall biases and subjective impressions may further reduce the data quality in some cases, as in population health surveys. In contrast, the survey design and administration itself may be unduly challenging. While EHRs, on the other hand, are accurate, wide-ranging, and more cost-effective, the collection and analysis of the data they contain requires sophisticated programs and deep knowledge of bioinformatics.
Benefits of RWD
Real-World Effectiveness
National health networks often contain vast amounts of data spanning the entire lifetime of millions of people. The fact that this is held in several different databases has been a stumbling block earlier. Currently, collaborative initiatives aim to collate the data in each of these databanks to exploit the statistical power of the large numbers of records and observations to improve the understanding of health and disease.
As the focus is on the interoperability of EHRs, hospitals, and clinics are developing integrated EHR systems to facilitate care across state and national boundaries. These quickly respond to such changes by updating the records electronically and instantaneously across all servers concerned. Such unified detailed data systems may be a treasure trove for understanding how well and how broadly various interventions work under real-world circumstances.
The increasing use of EHRs has made the collection of post-approval clinical data more widespread, accurate, and extended over a longer period. Analyzing such data could help understand which patients should get certain medications and how a given drug actually affects health in the real-world setting.
Currently, RWD, being observational, is considered supportive in nature, lower in the hierarchy of evidence than RCT data. No matter how strong the association, RWE is not thought to provide causal evidence that can be used as a basis for practice guidelines or regulatory approval. But slowly, researchers are waking up to understand that such observational data can fill in important RCT data gaps.
For instance, RWD can help understand how well heterogeneous populations that do not fulfill ideal eligibility criteria for a medication or product tolerate it or how well it works outside these preset criteria. This is how a clinical practice operates, and thus RWD provides a unique opportunity to gather a broad picture of drug efficacy, safety, and tolerability under less controlled conditions.
As this unique strength of RWD is better understood, RWD may help reveal true comparisons between how well different treatments work, how they affect biomarkers, how well they are accepted and used by doctors and patients, and their actual cost to the patient and safety concerns. Without the need for the scale of expenditure required for a typical RCT, RWE may bridge these crucial gaps more efficiently and provide post-marketing surveillance data in a shorter time than an RCT.
This can be made more accessible by the collaborative development of common guidelines on how such RWD should be collected, stored, managed, shared, and used in different settings. This will provide much-needed standardization of RWD, furthering its use as a source of data for RWE on comparative drug analyses and influencing healthcare coverage and payment.
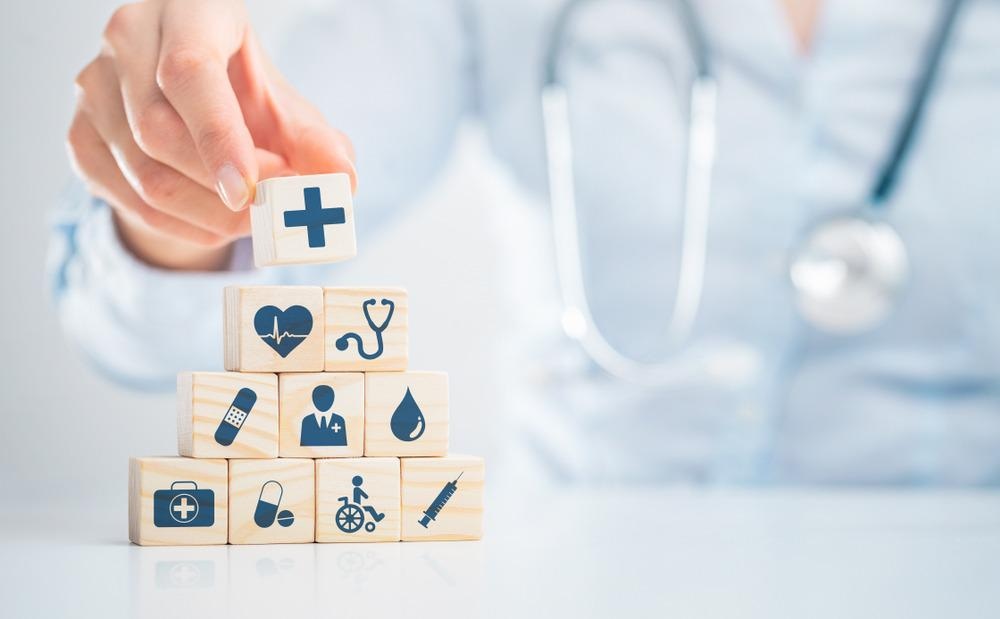
Image Credit: REDPIXEL.PL/Shutterstock.com
Drug Repurposing
Another vital area where RWE could play a major role is in providing the underpinnings for off-label uses of medications by showing the safety and effectiveness of their use in such situations and thus facilitating the expansion of the drug indications to include other uses. This is especially true when RCTs are scarce or small, but RWD is plentiful on the use and effectiveness of a drug off-label.
Safety Evaluation
The risk-benefit ratio of a medication is a central part of safety assessment. Regulatory agencies thus focus on identifying, tracking, and minimizing medication- and product-associated risks. Recent research in this area suggests using RWD to simulate an RCT in situations when the latter would be impossible, or at least to validate the utility of RWE by carrying out an RCT using RWD.
Such clinical trial simulations (CTS) are likely to be more realistic in their population base. Moreover, causal relationships can be estimated from such trials. It is also possible to link EHRs with other databanks to enlarge the field of study while exploring the correlations between drugs and their response phenotypes, or drugs vs. gene-driven responses.
Vast amounts of RWD are pouring in from wearables, mobiles, and other devices to measure health parameters. Biobanks also offer large amounts of 'omics data, which should be integrated into the same cycle as other data.
Simultaneously, bioinformatics technology is progressing rapidly to provide detailed and advanced analytic programs to pick out valuable patterns from such data that can help refine the drug development and approval process.
How Big Data Could Transform The Health Care Industry
What Lies Ahead for RWD?
Looking ahead, many challenges remain. Privacy of patient data is foremost, along with the ability to integrate data from different sources, delimit the bounds of the effectiveness of RWD, and develop reproducible and accurate analytic methods for RWD.
For healthcare to benefit maximally from RWD, it is necessary to frame guidelines for the optimal collection, documentation or representation, messaging, and structure of such data, finally culminating in its analysis. Standardized criteria must be set out, and further education provided on how to ensure that RWD yields the right kind of evidence, whether negative or positive and how to share such data for the common benefit.
Currently, most researchers ignore this potentially rich source of information. If this attitude changes and scientists begin to take RWE into consideration when evaluating healthcare interventions, many changes could occur in the way drugs are approved. RWD could also be crucial in shaping public healthcare, training artificial intelligence (AI) to help make clinical decisions, and streamlining clinical protocols.
Many researchers have established best practices to reap RWE from the wealth of RWD available today. This includes collaborative processes essential to prevent the omission of large data sources because of proprietary restrictions. Similarly, data entry at the source must be detailed and accurate, which can be assured only by providing incentives since the operator does not otherwise benefit from this work.
Natural language processing utilizes unstructured but very significant medical language, while flexible platforms allow multiple solutions to be implemented. Diverse sources must be used to ensure comprehensive use of RWD in the training of AI, especially in clinical management and during drug approval.
Equitable development must also be ensured during the use of RWD to generate RWE that is generalizable worldwide. The patients who ultimately contribute the data must be protected from privacy breaches and benefit from the evolution of better clinical practices and care, greater efficiency of operations involving the patient, and newer therapies.
"Practices that facilitate the RWD life cycle are poorly understood by healthcare professionals. Organizations must adopt new practices to realize full value from RWD and expand resulting capabilities."
References:
Further Reading