The COVID-19 epidemic, which began late December 2019 in Wuhan, China, and rapidly spread throughout the world, was accompanied by unprecedented releases of reported case data. Now, researchers propose combining phenomenological descriptions with epidemiological dynamics for an entirely new perspective on these data.
According to the World Health Organization (WHO) database, the first cases in Japan date back to January 14, in Italy to January 29, in France to January 24, 2020, etc. Since then, unprecedented data is available at institutions (such as the WHO and the Johns Hopkins University) for public information and use.
Understanding the information in this data may throw open new questions and challenges for the scientific community. To describe the epidemiological dynamics of this data, three researchers from University Bordeaux, CNRS, and University Grenoble Alpes, France, built a method using a phenomenological model and regularized data.
After comparing the various instantaneous reproductive numbers, they concluded that the dynamics of COVID-19 are primarily influenced by dynamics of social interaction (contact between individuals). They obtained an instantaneous reproduction number that stays below 3.5 from the early beginning of the epidemic.
Based on their findings, the researchers interestingly conclude that it is sufficient to vaccinate 71% of the population in each state or country (as considered in this study).
"Therefore assuming the vaccines will remain efficient against the new variants, and to be more confident it is sufficient to vaccinate 75 − 80% to get rid of COVID-19 in each state or country," noted the researchers, hoping then subsequently the people may go back to normal. This work is published on the preprint server medRxiv. It is to be noted that the preprint reports are not peer-reviewed and should not be used to guide clinical practice.
Flow chart for the model.

This news article was a review of a preliminary scientific report that had not undergone peer-review at the time of publication. Since its initial publication, the scientific report has now been peer reviewed and accepted for publication in a Scientific Journal. Links to the preliminary and peer-reviewed reports are available in the Sources section at the bottom of this article. View Sources
For this study, the researchers used the reported case data for eight different geographic areas: the state of California, France, India, Israel, Japan, Peru, Spain, UK. Except for the California state, for which they used data from the COVID tracking project, they took the rest of the reported case data from the WHO database.
They found that the phenomenological model applied to the COVID-19 data allowed them to handle the multiple epidemic waves and fit the data well for the eight geographic areas considered in this study.
"We expect that a number of different phenomenological models could be employed for the same purpose; however, our method has the advantage of involving a limited number of parameters."
As noted by the researchers, forecasting the propagation of the epidemic is, in particular, a key challenge in infectious disease epidemiology. Modeling efforts are employed to analyze and predict the outbreak and deduce the epidemic's transmission rate. This information can help epidemiologists, scientists, and importantly medical doctors and healthcare decision-makers.
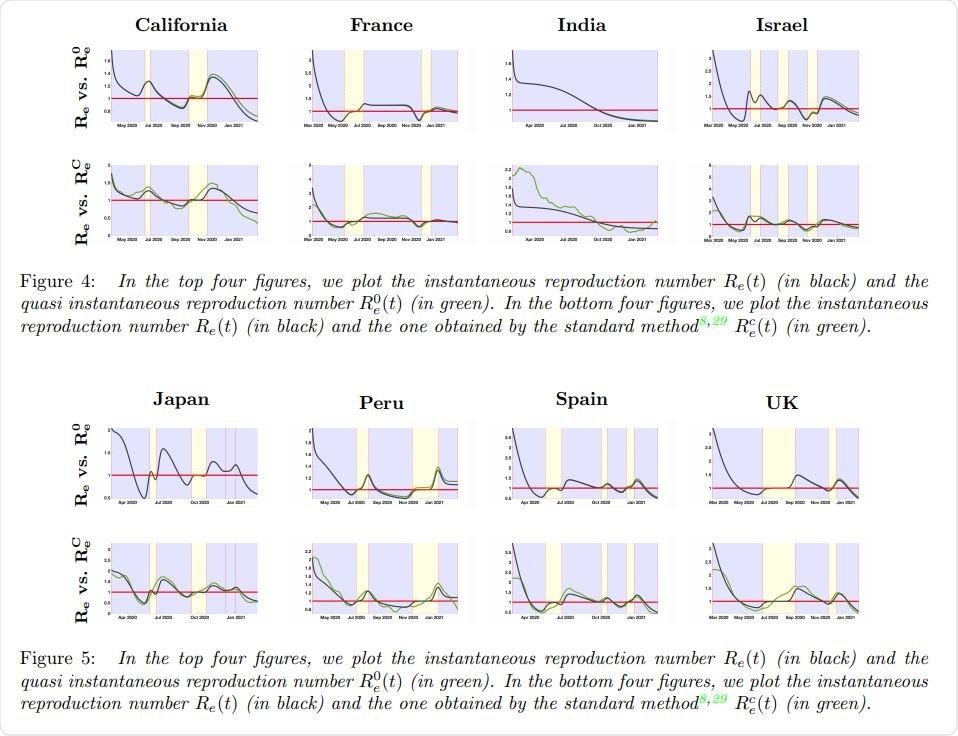
One of the most crucial challenges of an epidemic is understanding the transmission rate - the fraction of all possible contacts between susceptible and infected that effectively result in a new infection per unit of time. It depends on many factors, such as (i) the coefficient of susceptibility, (ii) the coefficient of virulence, (iii) the number of contacts per unit of time, (iv) and environmental conductivity.
In this study, the researchers attempted a novel method to understand and reconstruct the transmission rate from the data by using the model - without choosing a predefined function for the transmission rate, which was how the standard approaches were.
However, the researchers used an approach used in the early 70s (by London and Yorke), who used a discrete-time approximation model and discussed the time-dependent rate of transmission in the context of measles, chickenpox, and mumps.
Our analysis shows that it is hopeless to estimate the ascertainment rate using time series of cumulative cases only. Indeed, a time-dependent transmission can be computed for any reasonable value of this rate and perfectly fits the data. The same is true for other parameters of the mathematical model: the average duration of the non-infectious incubation period, the average duration of the infectious incubation period, the average duration of the symptomatic infectious period. Therefore, these parameters have to be estimated by statistical methods on samples of a different nature, like for the Diamond Princess cruise ship and the French aircraft carrier Charles de Gaulle.
Based on recent studies where an explicit formula and algorithms were used to reconstruct the transmission rate, the researchers understood that the regularization of the data is a complex problem. Therefore, reconstructing a meaningful time-dependent transmission rate is crucial, and they presented a new method to compute it from cumulative reported case data.
"While the use of a predefined transmission rate τ (t) as a function of time can lead to very nice fits of the data, here we are looking for a more intrinsic relationship between the data and the transmission rate."
In the paper, to compute the transmission rate directly from the data, they described their method in a two-step procedure: Firstly, we use a phenomenological model to describe the data and extract the general trend of the epidemic dynamics while removing the insignificant noise. Secondly, we derive an explicit relationship between the phenomenological model and the transmission rate.
Notably, the researchers reported that the social changes in the population have a more substantial impact on the propagation of the disease than the pure epidemiological dynamics while proposing a method to quantify these changes.
On predicting the future behavior of an epidemic, the researchers compared with the popular and standard method of framework (for the effective reproductive number), they observed their new indicators performed better near the beginning of the epidemic and close to the last data point, and were less variable in time.

This news article was a review of a preliminary scientific report that had not undergone peer-review at the time of publication. Since its initial publication, the scientific report has now been peer reviewed and accepted for publication in a Scientific Journal. Links to the preliminary and peer-reviewed reports are available in the Sources section at the bottom of this article. View Sources
Article Revisions
- Apr 10 2023 - The preprint preliminary research paper that this article was based upon was accepted for publication in a peer-reviewed Scientific Journal. This article was edited accordingly to include a link to the final peer-reviewed paper, now shown in the sources section.