In a recent study published in the journal Science Advances, researchers described a deep learning (DL)-based reconstruction framework to accelerate brain magnetic resonance imaging (MRI) at 0.055 tesla (T).
MRI is an invaluable imaging modality due to its non-invasive, non-ionizing, quantitative, three-dimensional (3D), and multiparametric nature. Research has focused on high-field (1.5 T or 3 T) MRI, commonly used for diagnosis and biomedical applications. Nevertheless, these high-field scanners are rare, with uneven global distribution.
As such, acute care facilities, trauma centers, and community and pediatric clinics can hardly access these scanners. There have been intensive efforts to develop affordable scanners at ultralow-field (ULF) strengths (< 0.1 T). Studies have been successful in implementing neuroimaging protocols on such ULF scanners. Moreover, analytical and DL methods eliminate the need for radio frequency shielding rooms.
Nevertheless, the lower signal-to-noise ratio (SNR) at ULF may undermine its clinical value, limiting widespread adoption. Further, nearly all ULF MRI developments rely on conventional image reconstruction methods from high-field MRI, compromising the usefulness of ULF MRI. Therefore, exploring alternative approaches to image reconstruction is necessary to improve the quality and speed of ULF MRI.
Study: Deep learning enabled fast 3D brain MRI at 0.055 tesla. Image Credit: ILevi / Shutterstock
The study and findings
The present study described a DL-enabled framework for rapid brain MRI at ULF. The researchers optimized and implemented T1- (T1W) and T2-weighted (T2W) protocols on a 0.055 T MRI scanner at 3 mm isotropic resolution. The scanner used a samarium-cobalt magnet and DL (to cancel electromagnetic interference) without shielding cages. SNR was increased by using a 3D fast spin echo sequence.
Data were obtained with a single number of excitation and 2D partial Fourier (PF) sampling in superior-inferior and left-right directions. The scan time was 2.5 minutes for T1W and 3.2 minutes for T2W. The team implemented a 3D DL PF super-resolution (SR) model to reconstruct ULF data, using a single low-resolution PF-sampled 3D image as the input.
The model was trained using under-sampled low-resolution 3D input image data (low SNR, 2D PF sampling, and 3-mm isotropic resolution) and high-resolution 3D target image data (high SNR and 1.5-mm isotropic resolution). Models were tested using synthetic ULF data simulated from large-scale public 3 T MRI data from the Human Connectome Project (HCP) consortium.
The PF-SR model reduced PF-related artifacts (blurring and ringing) and noise. Further, there was a substantial enhancement in spatial resolution. When ULF data were reconstructed using the conventional non-DL method, PF-SR outperformed the non-DL method in noise reduction and reconstruction of anatomical structures.
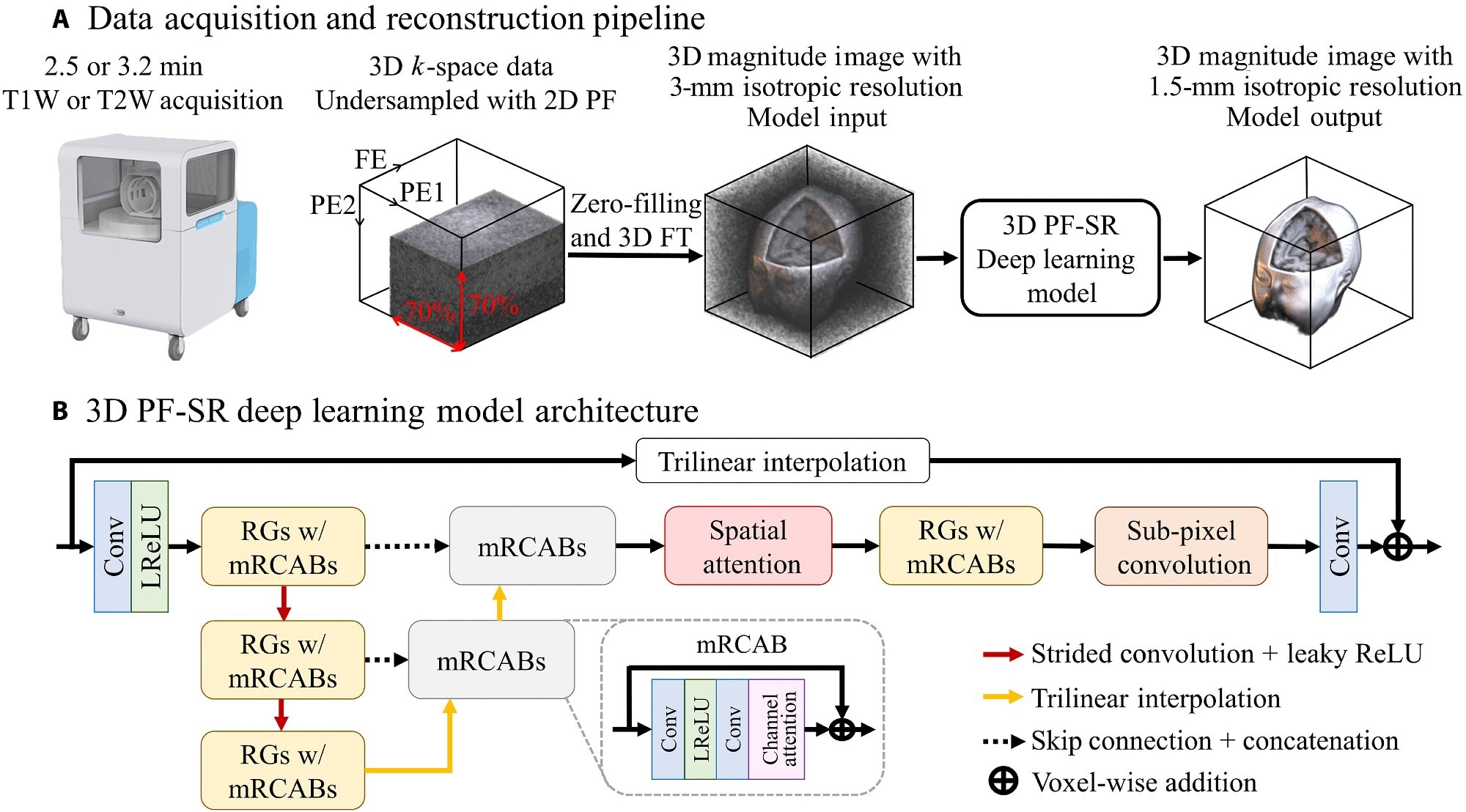
Data acquisition and DL reconstruction pipeline for fast ULF isotropic 3D brain MRI at 0.055 T. (A) Unlike typical ULF scans that acquire multiple NEXs, the proposed acquisition scheme acquires a single NEX, together with 2D PF sampling of a PF fraction of 0.7 in each of the two PE directions, in the FSE protocols for both T1W and T2W contrasts. The scan time is 2.5 and 3.2 min for T1W and T2W contrasts, respectively. The 3D data are then reconstructed by an end-to-end 3D DL model, which learns a residue between the high-resolution image and the interpolated low-resolution image. (B) The overall architecture of the DL 3D PF-SR reconstruction model. It is composed of residual groups (RGs) with modified residual channel attention blocks (mRCABs), multiscale feature extraction, spatial attention, and subpixel convolution. 3D convolution layer allows the effective extraction of 3D brain structural features. Multiscale feature extraction learns local features at the top-scale level and semiglobal features at the middle- to bottom-scale level. Spatial attention exploits the interspatial relationships by modulating the extracted features, which are then upsampled to the high-resolution space via subpixel convolution and transformed into a 3D image residue through a convolution layer. The final 3D image is formed by voxel-wise addition of the 3D image residue and the interpolated low-resolution image.
PF-SR consistently had a lower normalized root mean square error and higher structural similarity index than the non-DL method. Next, the team recruited 15 healthy individuals aged 25-69 and acquired their brain images on the 0.055 T MRI scanner. Data were reconstructed using PF-SR as well as the non-DL method. Additionally, subjects were scanned on a standard 3 T MRI scanner for reference.
The researchers observed that noise and PF-related artifacts were effectively reduced with PF-SR and spatial resolution was enhanced relative to the non-DL method. PF-SR delineated cerebrospinal fluid and white/grey matter. Structures recovered in PF-SR had higher clarity than non-DL and were consistent with the 3 T reference.
Moreover, in older subjects, PF-SR revealed aging-related atrophy in line with the 3 T reference. On the other hand, the non-DL method produced high noise and resulted in the loss of structural details. Finally, a reproducibility test performed on one individual at different head positions during two sessions demonstrated the robustness of PF-SR.
Conclusions
In sum, the authors achieved fast, high-quality whole-brain ULF MRI T through an integrated PF sampling acquisition and DL reconstruction framework. This approach considerably reduced the scan time of T1W and T2W protocols. The DL PF-SR model effectively reduced noise and PF-related blurring and ringing and improved spatial resolution.
The team evaluated model performance on different training sample sizes, i.e., 25%, 50%, and 100% of the training data. The least fluctuating training loss occurred when the model was trained on 100% of the data. The researchers also demonstrated that PF-SR could recover brain lesions; however, further evaluation is necessary to determine/validate the sensitivity and specificity of PF-SR.