Professor Frankel from Imperial College London speaks to News-Medical about his latest research that investigated whether AI could be used to predict infection outcomes.
What inspired your research into infections?
I got interested in infectious biology very early during my studies; it is very common that teachers and lecturers are the source of inspiration and this was true for me as well; I started my research as a Ph.D. student at the Hebrew University of Jerusalem studying infection with the Picornavirus Theiler's Murine Encephalomyelitis Virus (TMEV), as a model for MS.
After my Ph.D. training, I moved to Stanford University for my postdoc where I started to work on bacterial infections (E. coli and Salmonella), and from there I got fully hooked on bacterial pathogenesis.
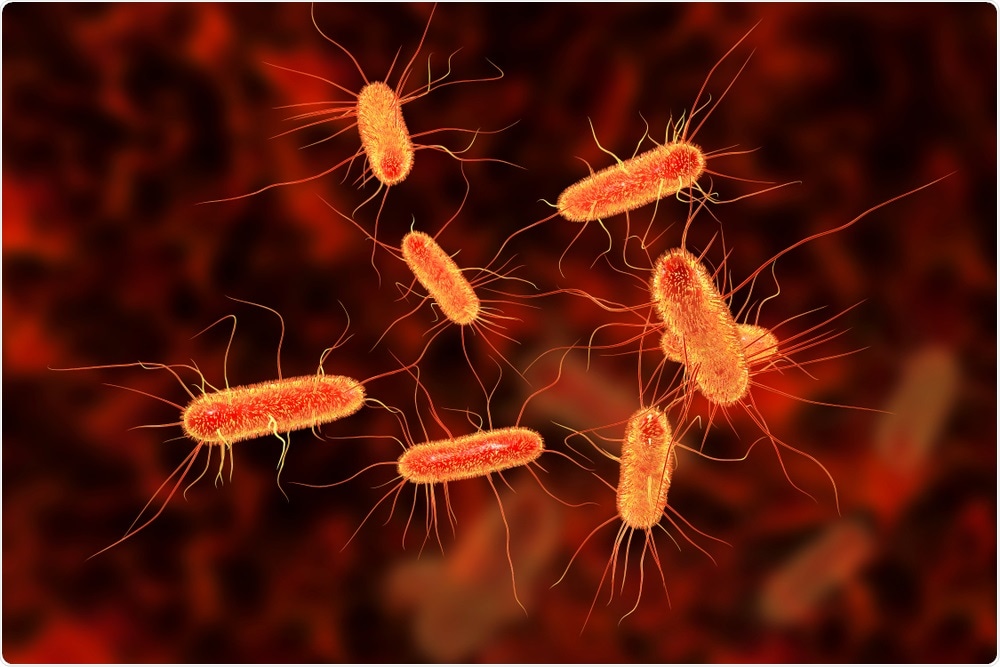
E. Coli. Image Credit: Kateryna Kon/Shutterstock.com
How do bacteria cause infections?
Disease-causing bacteria employ multiple mechanisms and devices to cause infection. The key principles of pathogenesis are colonization, multiplication, and evasion of host immune responses. While some pathogens remain extracellular, others colonize by invading human cells.
An efficient way to get hold of the host and cause disease is to use colonization factors, such as fimbrial adhesins (used for example by enterotoxigenic E. coli or Vibrio cholerae) and elaborate toxins that cause diarrhea.
Alternatively, several common enteric pathogens (for example enteropathogenic E. coli, Salmonella, and Shigella) use nano-syringes (called type III secretion systems) to inject bacterial proteins, known as effectors, into human cells; these effectors facilitate colonization, enabling multiplication (either intra- or extra-cellularly) and subversion of innate immune responses. This gives the pathogen a window of opportunity to survive in the host before adaptive immune responses (or treatment with antibiotics) clear the infection.
Why have studies so far only focused on single effectors as opposed to multiple ones? What are some of the limitations of this?
Traditionally, we have been studying one effector at a time in vitro (using cell biology, protein binding assays, and biochemical/structural methodologies), to investigate their mechanism of action, the host cell protein/s they bind or modify, and the signaling pathways they highjack; it is common that once the in vitro role of an effector is known, its function in vivo is tested.
While in previous years there were some technical constraints in generating multiple gene deletions in a single bacterium, today, this is not really an issue. The fact that most studies are done on single effectors is more due to the current convention than to technical limitations.
Can you describe how you carried out your latest research into infections?
My lab studies infections with the enteric human pathogens enteropathogenic and enterohaemorrhagic E. coli (EPEC and EHEC respectively); however, as EPEC is a human-restricted pathogen, mice are inherently resistant to this pathogen. As our aim is to study infection in the context of the endogenous gut microbiota and immune responses, we need to use animal models.
Accordingly, we are lucky we can use Citrobacter rodentium, which is a mouse-restricted pathogen equivalent to EPEC. EPEC and C. rodentium share the same infection strategy, including the nano-syringe and effectors; while the prototype human EPEC strain E2348/69 injects 21 effectors into intestinal epithelial cells (IECs), C. rodentium injects 31 effectors, which include the 21 effectors used by EPEC.
Importantly, over many years it has been shown that deletion of many effectors individually had little impact on the ability of the pathogen to infect the host. The original aim of this study was to determine what is the minimal number of virulence factors that could sustain the infection, using C. rodentium as a model. While pursuing this aim we developed the hypothesis that rather than working individually, the effectors form a robust network, which could tolerate significant perturbation.
To test this hypothesis, we perturbed the network by sequentially removing nodes (effectors), from two different starting points; in the first (pathway 1) we took an unbiased approach, deleting effector genes in a random order, in the second (pathway 2) we deleted genes encoding effectors that are thought to specifically target innate immune processes in IECs. Each intermediate mutant was tested for its ability to colonize mice above a 107 colony-forming counts / g of stool threshold.
Once we reached the robustness limits in pathways 1 and 2 (i.e. further effector mutants failing to colonize above the threshold), we use proteomics and bioinformatics to study how, compared to the wild type, these two synthetic C. rodentium strains affect cellular processes in IECs. We also characterized the type of immune response they triggered. In addition, we removed the 10 extra effectors found in C. rodentium compared to EPEC and tested the impact on the infection outcome.
As in the process of sequential effector deletion we have generated over 100 intermediate mutants which were studied in vivo, we combined these data with our knowledge of the effector function, to train a machine model with the aim of using AI to predict infection outcomes. We used the AI model to score the 16,489,545 possible mutant combinations lacking up to 9 effectors.
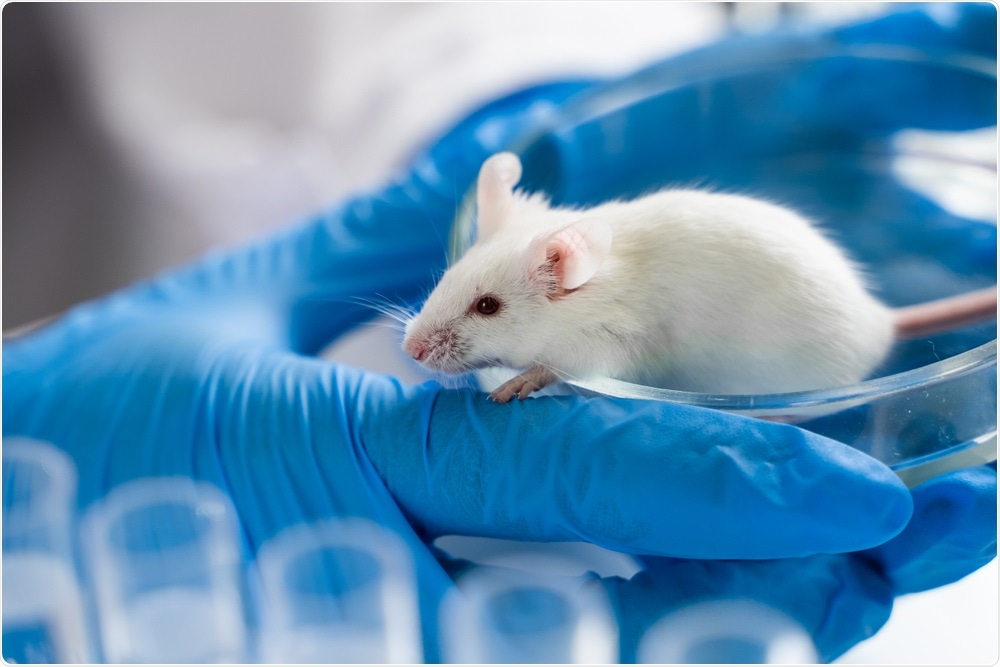
Mouse Model. Image Credit: Egoreichenkov Evgenii/Shutterstock.com
What did you discover?
We found that the effector network is highly robust, as it can tolerate contraction by 60%. In pathway 1, a mutant expressing only 12 of the 31 effectors (a strain we named CR14) maintained the ability to colonize, while in pathway 2 the pathogen tolerated deletion of 10 effectors known to affect key innate immune responses (a strain we named CRi9). At this stage, we ended with three distinct functional effector networks (wild type, CR14, and CRi9).
Interestingly, while expressing distinct effector networks, these strains induced conserved infection signatures, which included shifting of ATP production from the mitochondria to the cytosol (via aerobic glycolysis) and tissue damage repair responses (i.e. proliferation of IECs). Conversely, infection with these strains triggered vastly different immune responses (i.e. CR14 and CRi9 induced a significant increase in the secretion of mucosal GMCSF and TNF/IL-22/IL-17/IFNgamma, respectively).
Yet, the host was able to clear the infections and develop long-term protective immunity. This shows that while the pathogen encodes a robust effector network, host immunity is similarly flexible and able to cope with vastly different infection strategies.
We found that the EPEC effector network did not enable efficient C. rodentium infection, suggesting that the composition of the effectors plays a role in host specificity.
Finally, the best colonization failure predictions of five and nine effector deletions, predicted by the AI model, were tested in mice. Remarkably, these mutants failed to colonize. Importantly, the prediction of five effector deletions included four effectors that have functional homologs in Shigella, increasing the confidence in the AI.
How could your latest research help to predict the outcomes of infections?
It is important to remember this is just the beginning of a long path for using AI to predict the outcome of infections with bacterial pathogens using an injection of effectors as a colonization strategy.
Thus far the AI model was able to predict the outcomes of infection with a model pathogen (C. rodentium) expressing different effector networks. As it is impossible to test in the lab all the possible networks that 31 effectors can form, employing an AI model is the only practical approach to studying biological systems of this complexity.
The AI allows us to focus on creating the most relevant combinations of effectors and learn from them how bacteria are counteracted by our immune system. These combinations would not be obvious from our experimental results alone, opening up the possibility of using AI to predict infection outcomes.
What role did artificial intelligence (AI) play in your research?
The AI component was instrumental in ‘making sense’ of data collected in our, and many other labs, over many years. It enabled us, for the first time, to study effectors as networks.
After more than 20 years of experience in working with effectors, I would not be able to duplicate the predictions made by the AI; in fact, when I first saw the predictions, my gut feeling was that they were wrong. Obviously, I was pleased to be proven wrong, but at the same time, it put my knowledge in a new perspective.
It is important note that the AI part of the project was done by a PhD student, Elena Núñez-Berrueco, who is supervised by Prof. Alfonso Rodríguez-Patón from the Laboratorio de Inteligencia Artificial, Departamento de Inteligencia Artificial, Universidad Politécnica de Madrid, Spain.
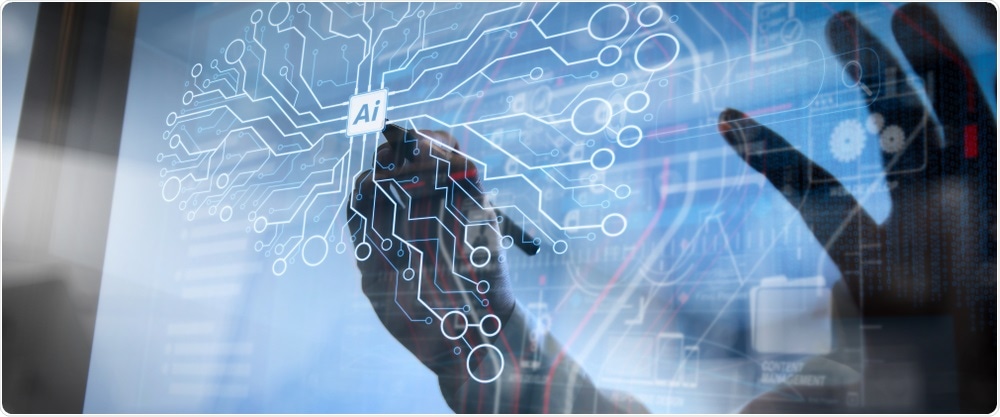
Artificial Intelligence. Image Credit: everything possible/Shutterstock.com
What other areas within immunology and diseases could you apply this knowledge to?
Our study shows that we can predict how a cell will respond when attacked by different combinations of bacterial effector networks.
The research will help us to better understand how cells, the immune system, and bacteria interact, and we can apply this knowledge to diseases like cancer and inflammatory bowel disease where bacteria in the gut play an important role.
Do you believe that with further knowledge of effector networks we could help to design treatments that can disrupt their effects?
Our results mean that in the future, using AI and synthetic biology, we should be able to work out which cell functions are essential during infection, enabling us to find ways to fight the infection not by killing the pathogen with antibiotics, but instead by changing and improving our natural defense responses to infection.
We hope that further studies would allow us to build on these foundations and work out exactly how these effector proteins function, and how they work together to disrupt host cells. In the future, this enhanced understanding could lead to the development of new treatments.
What are the next steps in your research?
The most urgent next step is to gather more data to train the AI model. Moreover, all the measurements we have done in this study were point-in-time, at 8 days post-infection. The next step is to conduct a time course study and investigate how the host is responding to C. rodentium expressing different effector networks over time (from the inception of the infection to clearance).
We then need to study how the effectors work as a group at a molecular, mechanistic, level, this is the most challenging aspect of future research. Finally, we should apply the pipeline to study other pathogens that use their own set of effectors for infection (for example Shigella or Salmonella, which are invasive pathogens).
Where can readers find more information?
https://science.sciencemag.org/content/371/6534/eabc9531
Who funded this research?
This project was supported by a Wellcome Investigator Award.
About Professor Frankel
Professor Frankel is a Professor of Molecular Pathogenesis at the MRC Centre for Bacteriology and Infection at Imperial College London.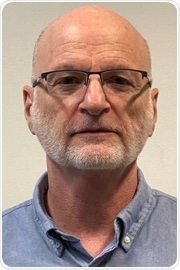
His lab has identified the extension to the molecular syringe used by EPEC and set up the foundations for the Citrobacter rodentium model. Our research is supported by the Wellcome Trust, the MRC, and the Royal Society.