Please can you give an overview of the current drug discovery paradigm? In what ways do you think it needs to be leaner?
With the current drug discovery paradigm, it takes up to 15 years to translate an idea, such as hypothesizing a certain protein is important in a disease and testing this with targeting the protein with a drug, all the way through to proof of concept. The drug has to be filed with the regulatory authorities, having done all the safety and efficacy testing. It's a long process and it's also very costly.
Estimates vary, but it's currently reckoned to cost over 1 billion dollars per drug. That’s partly because you have to pay for all the drugs that didn't make it. By the time you've taken a protein target and you've made a whole load of molecules that affect that target in the way you want them to, you've probably already spent a couple of years on it and then you have to take the best molecule through the appropriate safety testing in animals before proceeding to phase 1 testing in people.
Even if you get to phase 1 testing, where you are just giving it to human volunteers for the first time, not even patients, about 95% of the compounds at the stage then fail to make it all the way through to the market. So, you’ve only got a 5% success rate.
The odds are not good and, essentially, it is why so many companies have had to merge because, over time, this is becoming less and less sustainable as a business model.
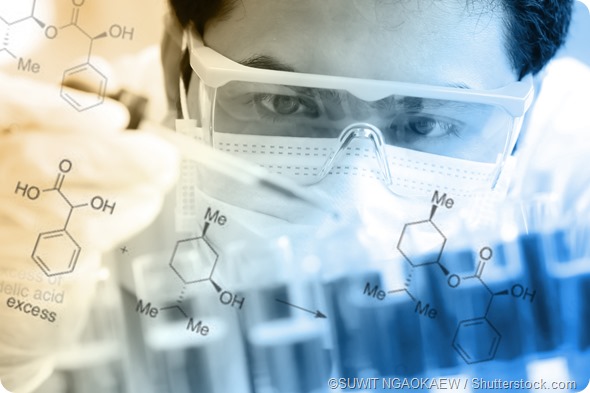
Why do so many of the compounds fail?
One of the main reasons why compounds fail is that they actually don't work in the patients they are tested in. We may have picked the wrong target since although we've actually created a molecule that, for example, inhibits the activity of the protein in the disease, it may actually turn out that over activity of that e protein isn't as important as we thought it was in that disease.
The other reasons molecules fail now is primarily unexpected toxicity. You could take a molecule all the way through to phase 3 trials – the biggest trials in patients – and suddenly find that in your long-term, two-year carcinogenicity studies in animals, that you've got something that is quite cancer-provoking, so you have to stop development. Those are the two reasons: efficacy and toxicity.
Somehow, we've got to find a way of trying to increase the chances of success or making the process less costly and leaner.
What is deep learning and how do you think it could be used to improve the drug discovery paradigm?
You're average biomedical researcher is dealing with a huge amount of new information every day. You're getting over 10,000 new publications uploaded on a daily basis worldwide across all the biomedical journals and databases, so there is no way that one researcher can actually even access, let alone read all these huge amounts of data.
What we have to do is use deep learning to be able to help us ingest all that data and do some initial deep triage of that data to surface new therapeutic opportunities. It’s meant to provide insight for our biologists and chemists, so they can actually deal with a usable amount of information.
How important is it to pick the right target in drug discovery? Could artificial intelligence (AI) be used to aid this process?
Picking the right target is absolutely fundamental. I mentioned that over half of failures of new medicines in development are due to us picking the wrong target. Even if we just doubled our success rate at picking the right target, it would have a huge impact on the development of new medicines for people.
One of the reasons, and why I think artificial intelligence could be really important in helping us do that, is that, quite often, there may be some data buried in the literature that tells us a target is really good. Equally valuable is the fact that there may be something buried in the literature that tells you a target is not good.
It's much harder to publish negative data than positive data. That target invalidation can be just as valuable as a target validation. It's really about being able to tap into all that knowledge and look up all the facts. That would mean that our systems and other people's AI systems can really be much more effective at picking the right target.
Can you please give an overview of BenevolentBio?
What we're trying to do here at Benevolent is develop systems and processes that allow us to tap into the huge amount of public information and some propriety databases that we have paid to access and through that, generate hypotheses which our scientists can then triage.
We've got over ten times the amount of information in our database at present (and it’s growing) than we believe that companies such as Watson have. Bringing that and filtering the signals from the ‘noise’ to our experienced drug discovery chemists and biologists, means, firstly, we can reduce the amount of time we take to triage new hypotheses, and secondly, we can develop more predictive chemical tools to allow us to make better molecules and also to reposition existing molecules for new indication.
What impact do you think the use of AI in drug discovery could ultimately have for patients?
One of the first ways in which this would be really felt by patients is through the repurposing of existing drugs for new diseases.
Clearly, even though one could improve the process in a number of ways in terms of speeding up medicinal chemistry programs and potentially speeding up the amount of things such as toxicity trials etc., that is still going to take time. However, if we can take existing drugs, perhaps drugs which have gone off patent or are currently being marketed for another indication, and repurpose them in areas where there are high unmet medical needs, those drugs could go into that new indication in phase 2 in patients and you would know very quickly whether the drug worked or not.
Then the route to market or the route to being able to make the drug more broadly available to patients would be much more rapid because you don't have to go through all those earlier stages of toxicity, testing, phase 1 testing, volunteers and so on.
What’s your vision for BenevolentBio?
I want us to disrupt the drug discovery and development process and to look at each place on the drug discovery and development pipeline, so that we can be much better at getting the right target, much quicker at getting the right compound and much more confident that those compounds have the right characteristics which mean they will be safe and well tolerated.
Then we can go to the right patient population with the right dose, so we would have a much leaner, more successful process and be able to demonstrate the value of our AI technology.
What do you think the future holds for deep learning with regards to drug discovery?
I think we're only just scratching the surface at the moment. I think over the next couple of years, we'll start to see some major strides. Obviously, showing the effects on target identification takes some time with new molecules but, certainly, the repositioning effects should be seen in a few years.
I think there is great promise in terms of being able to really tap into a much broader chemical space, so we can have a much wider and more varied chemical palette to be able to pick the best molecules for drug discovery from.
Also, accelerating the ways in which we can look at the data and glean much more information from it can help us both in terms of patient selection for clinical trials and in terms of being able to pick up signals of efficacy and safety much earlier.
Where can readers find more information?
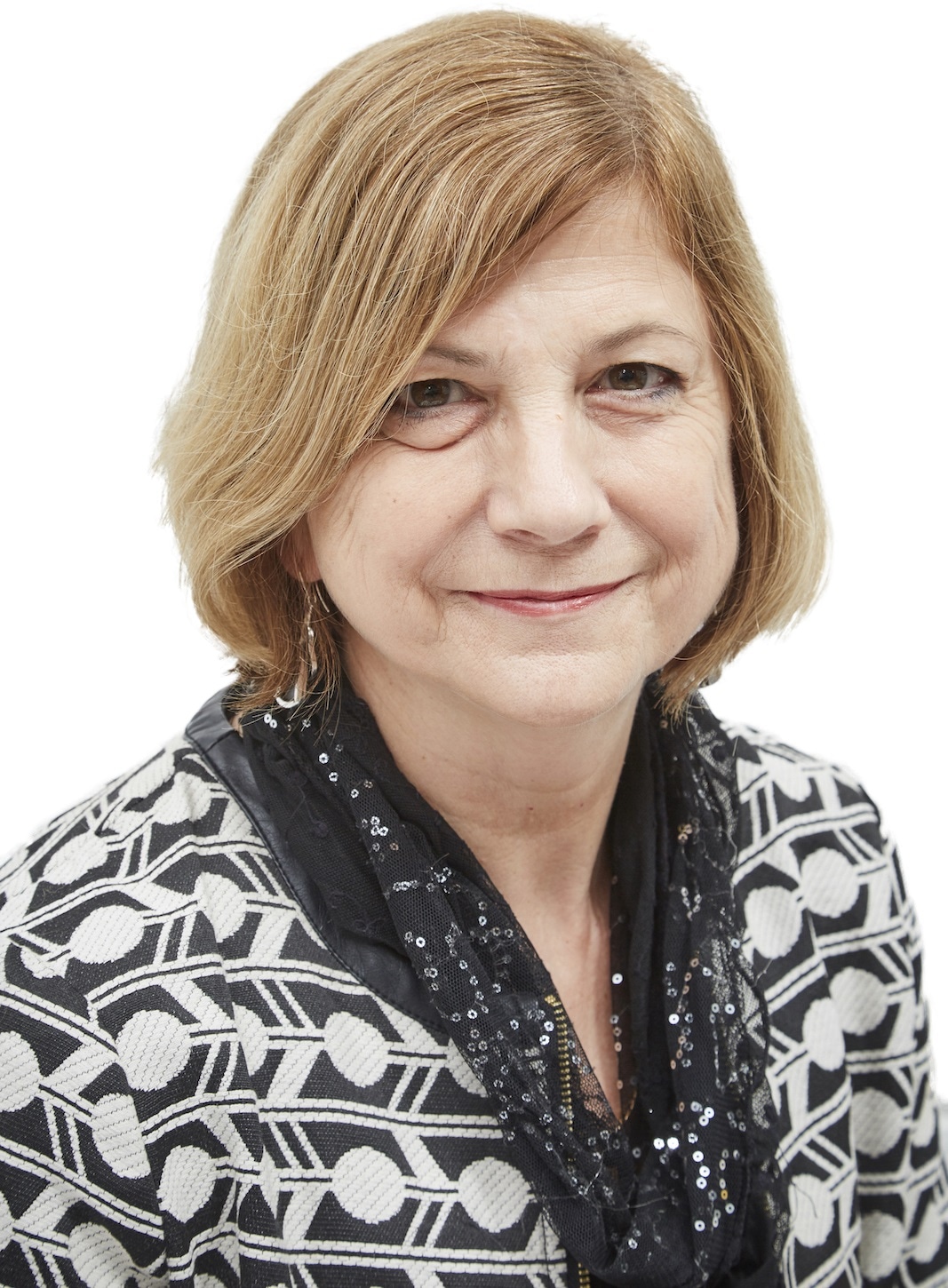
About Professor Jackie Hunter
Jackie is the CEO of BenevolentBio. She has held senior positions at global pharmaceutical organisations including GSK, Proximagen and OI Pharma Partners and joined BenevolentAI as CEO of BenevolentBio in 2016.
Jackie has a vast academic and business experience in the biomedical and pharmaceutical sectors.
She directs the application of BenevolentAI’s technology for drug development and give the company the insight it needs to operate its unique business model – one which sees it not only researching, but also developing the blueprint for new drugs.