A fundamental aspect of pandemic preparation is being able to predict viral evolution under dynamic immunological limitations. In viral diseases, immune recognition and evasion are linked, as these two properties can result in rapid evolution and alterations in the virulence of viruses.
Most observations made through pandemic surveillance are confined to forecasting observed mutations. Unfortunately, these efforts are limited in their ability to predict future mutational changes and are associated with biases resulting from sampling and epidemiological approaches.
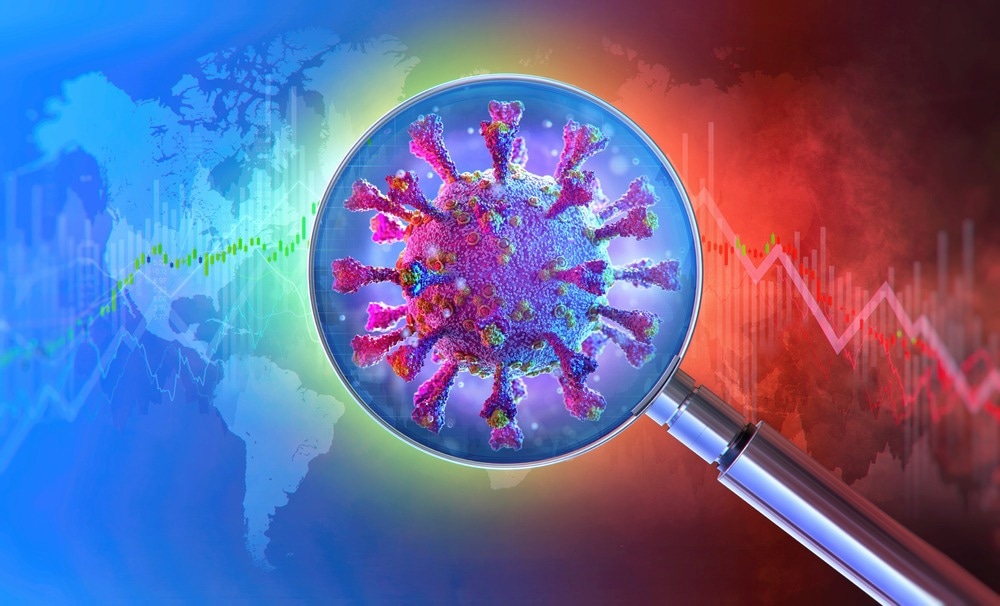
Study: Learning from pre-pandemic data to forecast viral antibody escape. Image Credit: Corona Borealis Studio / Shutterstock.com

*Important notice: bioRxiv publishes preliminary scientific reports that are not peer-reviewed and, therefore, should not be regarded as conclusive, guide clinical practice/health-related behavior, or treated as established information.
A new study published on the bioRxiv* preprint server reports early prediction of immune evasion using EVEscape, a biologically grounded model used in high-throughput experiments of antibody binding throughout the coronavirus disease 2019 (COVID-19) pandemic.
Background
Through the natural evolution of viruses, escape mutations are inevitable and often emerge when antibodies that restrict but do not hamper viral replication, impose selection pressure. Under these circumstances, a virus successfully evades the pressure by restoring its capacity to multiply more efficiently.
Such mutations influence the reinfection rates and duration of vaccine-induced immunity in populations. Thus, it is essential to anticipate potential mutations that will evade immunity with enough time to allow scientists to produce the most effective vaccines and therapeutics.
To quantify the viral escape potential that may have the most significant impact on human health, several experimental and computational methods have been applied. Although these techniques are efficient for predicting antibody evasion, they are cumbersome and unsuitable for investigating combinatorial mutagenesis.
Furthermore, these approaches do not rely on other crucial information for predicting antibody escape. Broad viral evolution may also include insufficient information about host-specific immunity.
To address these challenges, the researchers of the current study utilized EVEscape. This robust and modular method was developed for predicting viral antibody escape and can be employed at the onset of a pandemic for continual monitoring of risks posed by new variants. The framework of EVEscape captures evolutionary data, structural traits, and residue dissimilarities in an easy-to-interpret and biologically grounded approach.
EVEscape combines epistatic effects and, as a result, is extensible to variant combinations. This model is superior to existing methods and may be applied to a variety of viruses.
In the current study, the researchers utilized EVEscape for analysis of the severe acute respiratory syndrome coronavirus 2 (SARS-CoV-2), the virus responsible for COVID-19, as well as the human immunodeficiency virus (HIV), and the H1N1 virus that causes swine flu.
The most important aspect of EVEscape is that it provides an early warning of alarming mutations. This can prompt the immediate development of better vaccines, antibody therapies, and diagnostic techniques to mitigate transmission. Additionally, the information generated could be used to guide public health decisions and preparedness efforts, which would ultimately aid in curbing human and financial losses associated with future pandemics.
About the study
In the current study, multiple sequence alignments were produced for each viral protein. Crystal structures representing known structural states for each viral surface protein were selected. All heteroatoms and protein chains that were not present in the trimeric surface protein of the virus were excluded.
The protein data bank of the United States was searched to detect and identify antibody fingerprints for viral surface proteins. The primary variables identified for each viral mutational scan included protein fitness or antibody evasion.
Data on spike mutations and their deposition dates were extracted from the EpiCoV project database of the Global Initiative for Sharing All Influenza Data (GISAID). In addition to counts of combinations of mutations, the date of emergence, and Phylogenetic Assignment of Named Global Outbreak (PANGO) lineage, the scientists also determined the month of emergence for each mutation in the spike protein. SARS-CoV-2 consensus mutations for each PANGO branch were also retrieved.
EVEscape assesses the likelihood of a mutation to escape immune response based on the probabilities of a given mutation to maintain viral fitness, to occur in an antibody epitope, and to disrupt antibody binding, using information available early in a pandemic.
Study findings
EVEscape was used to analyze data from pre-pandemic sources including sequence likelihood projections from broad viral evolution, information on antibody accessibility from protein structures, and information on changes in binding interaction propensity from residue chemical characteristics.
A mutation's probability of inducing immune escape was a function of three probabilities, which included the likelihood of preserving fitness ('fitness' term), the likelihood of targeting an antibody epitope ('accessibility' term), and the likelihood of disrupting antibody binding ('dissimilarity' term).
A deep and unsupervised generative model of mutation effects was utilized to compute the fitness term. The accessibility term of a protein site was modeled using the negative of the weighted contact number calculated from viral protein structures, whereas the dissimilarity term was based on the difference in charge and hydrophobicity between the mutant and wildtype residues.
EVEscape anticipates antibody evasion
EVEscape surpassed deep unstructured sequence models and surface accessibility measurements regarding the quality of its top escape predictions across varied experimental techniques and datasets. Moreover, EVEscape captured 2.5 times more probable escape mutants, which amounted to 31% of the total measured. The overall precision of this approach was 2.7 times higher than the former techniques.
EVEscape could also effectively identify escape mutations in polyclonal patient sera. Over 50% of sera escape sites from patients infected with the original SARS-CoV-2 strain, as well as the Beta and Delta variants of concern (VOCs), accounted for the top 10% of EVEscape predictions.
EVEscape captures antibody footprints and escape potential. a) Precision-Recall of RBD DMS escape mutations (AUPRC reported compared to “null” model). b) EVEscape captures mutations that effect recognition by convalescent sera from patients infected with different VOCs. c) RBD site-averaged EVEscape predictions plotted against site-averaged Bloom DMS escape, with hue indicating known antibody footprints. d) RBD site-averaged EVEscape predictions (PDB: 7BNN). e) RBD sites of DMS escape mutants and of known antibody footprints (PDB: 7BNN).
Identification of least escapable non-neutralizing antibodies
As a result of the EVEscape predictions, escape mutations have been predicted at diverse epitope locations, with extensive coverage of antibody subclasses one through three and low coverage of the antibody subclass four.
Previous studies have reported that E484, K417, and L452 mutations are immunodominant sites in classes one and two. Convalescent patients' sera were dominated by antibodies of class two, followed by antibodies of classes three and one.
A cryptic epitope on the spike protein trimer is bound by class four antibodies when two of the three receptor-binding domains (RBDs) are in the 'up' configuration. SARS-CoV-1 and SARS-CoV-2 can be bound by these antibodies; however, their neutralization potentials are lower.
EVEscape predictions identify escape-resistant classes of antibodies. a-b) EVEscape scores of observed escape mutations cover diverse epitope regions across antibody classes including known immunodominant sites (E484, K417, L452) (PDB: 7BNN). c) EVEscape scores observed escape mutants from narrow antibodies and broad neutralizing antibodies higher than those from broad, non-neutralizing antibodies. Miniature boxplots within violin plots indicate median and interquartile range.
EVEscape forecasts pandemic and future mutations
The prevalence of VOC mutations in the top 25% of spike EVEscape predictions reflected their neutral or beneficial effects on general viral fitness, as well as immune evasion by SARS-CoV-2. Several of the remaining VOC mutations, including S375F and T376A, which are low among EVEscape predictions, are known to reduce fitness, including infectivity, and are frequently reversed.
By predicting VOC mutations, EVEscape demonstrated its ability to learn various viral fitness and immunological escape restrictions. Several VOCs have increased angiotensin-converting enzyme 2 (ACE2) affinity, which may contribute to their immune evasive characteristics.
The SARS-CoV-2 Delta and Omicron variants, which are known for their fitness and immune evasive capabilities, received the highest scores for random sequences with the same mutational depth. VOCs such as Omicron BA.4 are similar to sequences of random mutations at the same depth, as well as sequences composed only of mutations that are known to favor viral immune evasion.
EVEscape anticipates single mutations and strains observed in the SARS-CoV-2 pandemic. a) Site-averaged EVEscape scores on Spike structure (PDB: 7BNN) depict regions of high EVEscape scores (RB D, particularly the ACE2 binding region (RBM), and NTD). Spheres indicate sites with GISAID mutations observed more than 10K times. b) EVEscape mutation scores correspond to observed GISAID mutations in the RBD. c) Percentage of mutations in each EVEscape quartile seen >100 times in GISAID over time d) The majority of mutations in VOC strains are in the top quartile of Spike EVEscape predictions. e) BA.4 has a high EVEscape score compared to random samples of mutations at the same mutation depth. f) EVEscape z-scores increase throughout the pandemic, particularly for VOCs. Z-scores are relative to random combinations at the same mutation depth of single mutations seen over 1000 times in (GISAID. Note: EVEscape percentiles have been adjusted to consider only mutations that are a nucleotide distance of one away from Wuhan.
Conclusions
The EVEscape platform is capable of identifying the most concerning variants in the vast pool of available pandemic sequence data. This method provided predictions for all SARS-CoV-2 spike mutants, as well as strain-level forecasts for variants observed 1,000 times or more in GISAID. EVEscape will continue to be updated as new strains are identified.
Due to the generalizability of the framework across viruses, EVEscape can be applied to future pandemics. Furthermore, this system can be used to better understand and prepare for newer emerging communicable diseases.

*Important notice: bioRxiv publishes preliminary scientific reports that are not peer-reviewed and, therefore, should not be regarded as conclusive, guide clinical practice/health-related behavior, or treated as established information.