Feb 9 2017
Scientists from Mail.Ru Group, Insilico Medicine and MIPT have for the first time applied a generative neural network to create new pharmaceutical medicines with the desired characteristics. By using Generative Adversarial Networks (GANs) developed and trained to "invent" new molecular structures, there may soon be a dramatic reduction in the time and cost of searching for substances with potential medicinal properties. The researchers intend to use these technologies in the search for new medications within various areas from oncology to CVDs and even anti-infectives. The first results were submitted to Оncotarget in June 2016 and spent several months in review. Since that time, the group has made many improvements to the system and engaged with some of the leading pharmaceutical companies.
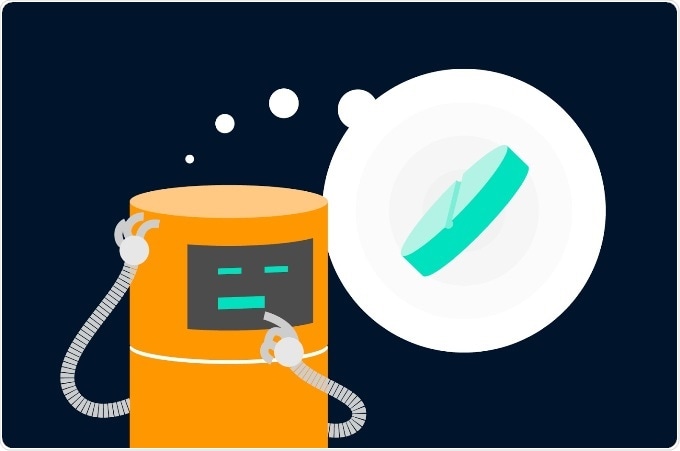
Currently, the inorganic molecule base contains hundreds of millions of substances, and only a small fraction of them are used in medicinal drugs. The pharmacological methods of making drugs generally have a hereditary nature. For example, pharmacologists might continue to research aspirin that has already been in use for many years, perhaps adding something into the compound to reduce side effects or increase efficiency, yet the substance still remains the same. Earlier this year, the scientists at Insilico Medicine demonstrated that it is possible to substantially narrow the search using deep neural networks. But now they have focused on a much more challenging question: Is there a chance to create conceptually new molecules with medicinal properties using the novel flavor of deep neural networks trained on millions of molecular structures?
Generative Adversarial Autoencoder (AAE) architecture, an extension of Generative Adversarial Networks, was taken as the basis, and compounds with known medicinal properties and efficient concentrations were used to train the system. Information on these types of compounds was input into the network, which was then adjusted so that the same data was acquired in the output. The network itself was made up of three structural elements: an encoder, decoder and discriminator, each of which had its own specific role in "cooperating" with the other two. The encoder worked with the decoder to compress and then restore information on the parent compound, while the discriminator helped make the compressed presentation more suitable for subsequent recovery. Once the network learned a wide swath of known molecules, the encoder and discriminator "switched off", and the network generated descriptions of the molecules on its own using the decoder.
Developing Generative Adversarial Networks that produce high-quality images based on textual inputs requires substantial expertise and lengthy training time on high-performance computing equipment. But with images and videos, humans can quickly perform quality control of the output. In biology, quality control cannot be performed by the human eye and a considerable number of validation experiments will be required to produce great molecules.
All the molecules are represented as "SMILEs", or graphical annotations of chemical substances that allow their structure to be restored. The standard registration taught in schools does not fit for network processing, but SMILEs do not do the job very well either, as they have a random length from one symbol to 200. Neural network training requires an equal description length for the vector. The "fingerprint" of a molecule will solve this task, as it contains complete information on the molecule. There are a lot of methods out there for making these fingerprints, but the researchers used the simplest binary one available consisting of 166 digits. They converted SMILEs into fingerprints and taught the network with them, after which the fingerprints of known medicinal compounds were input into the network. The network's job was to allocate inner neuron parameter weights so that the specified input created the specified output. This operation was then repeated many times, as this is how training with large quantities of data is performed. As a result, a "black box" capable of producing a specified output for the specified input was created, after which the developers removed the first layers, and the network generated the fingerprints by itself when the information was run through again. The scientists thus built "fingerprints" for all 72 million molecules, and then compared the network-generated fingerprints with the base. The molecules selected must potentially possess the specified qualities.
Andrei Kazennov, one of the authors of the study and an MIPT postgraduate who works at Insilico Medicine, comments:
We've created a neuronal network of the reproductive type, i.e. capable of producing objects similar to what it was trained on. We ultimately taught this network model to create new fingerprints based on specified properties.
The anticancer drug database was used to check the network. First the network was trained on one half of the medicinal compounds, and then checked on the other part. The purpose was to predict the compounds already known but not included in the training set. A total of 69 predicted compounds have been identified, and hundreds of molecules developed using a more powerful extension of the method are on the way.
According to one of the authors of the research, Alex Zhavoronkov, the founder of Insilico Medicine and international adjunct professor at MIPT:
Unlike the many other popular methods in deep learning, Generative Adversarial Networks (GANs) were proposed only recently, in 2014, by Ian Goodfellow and Yoshua Bengio’s group and scientists are still exploring its power in generating meaningful images, videos, works of art and even music. The pace of progress is accelerating and soon we are likely to see tremendous advances stemming from combinations of GANs with other methods. But everything that my groups are working on relates to extending human longevity, durability and increasing performance. When humans go to Mars, they will need the tools to be more resilient to all kinds of stress and be able to generate targeted medicine on demand. We will be the ones supplying these tools.
"GANs are very much the frontline of neuroscience. It is quite clear that they can be used for a much broader variety of tasks than the simple generation of images and music. We tried out this approach with bioinformatics and obtained great results," concludes Artur Kadurin, Mail.Ru Group lead programmer of the search optimizing team and Insilico Medicine independent science advisor.