People are constantly trying to find more ‘human’ ways to interact with technology. Could the same be achieved for how we approach our health too?
After the recent Deep Learning in Healthcare conference held in London, it is clear that technology has the capacity to transform healthcare as we know it.
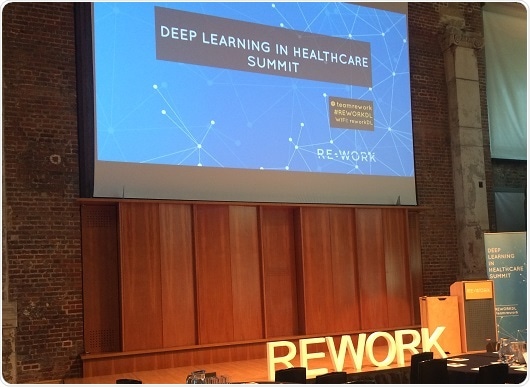
Deep learning (also known as machine learning or artificial intelligence) refers to a set of algorithms whereby a computational system is “trained” to process data by being tested on some information, which it then “learns” from. This results in a computer which has the ability to assimilate, process and present data to humans, eliminating work for us humans in the process.
Several speakers at the event described how deep learning can be used in diagnostic systems, including Oladimeji Farri, Senior Research Scientist from Philips, who described the system they have developed that could give a diagnosis based on a patient’s symptoms by drawing in information from the “signs and symptoms” sections of Wikipedia and using titles of the articles as the diagnosis.
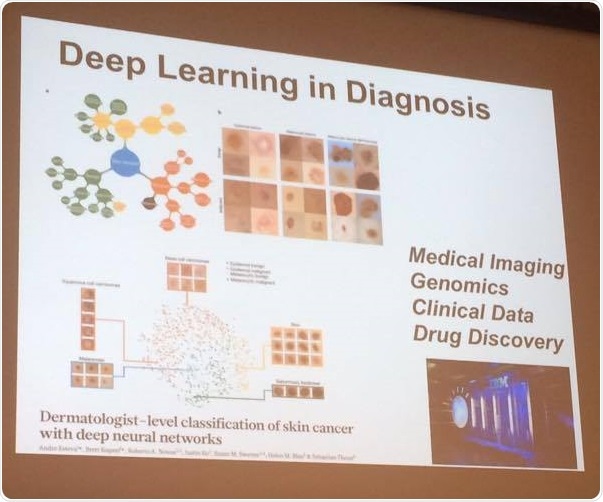
Viktor Kazakov introduced Skin Scanner – a convolutional neural network algorithm that can classify patients' skin diseases. Patients can submit a picture of an area of skin that is troubling them, then the system will visually scan it to match it to one of 23 skin disease classes that it has learned to recognize. The system has been shown to classify 67% of images by the correct disease category.
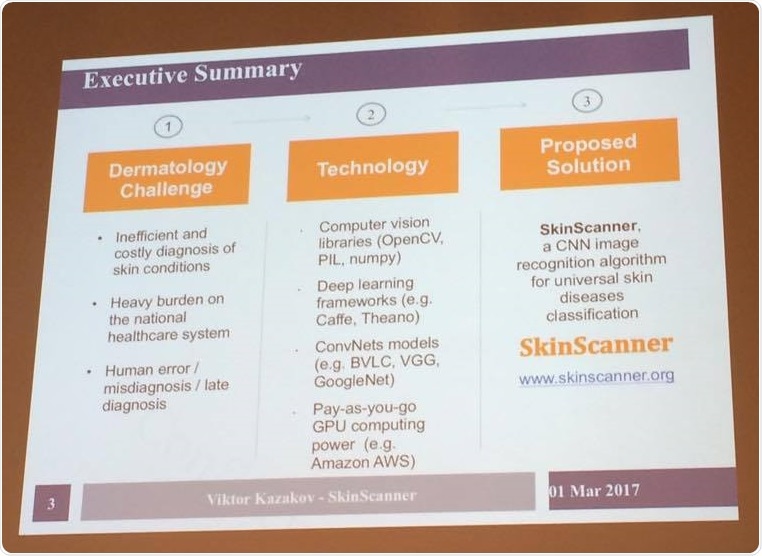
With the current lengthy drug discovery process resulting in a 95% fail rate, Polina Mamoshina, Research Scientist from Insilico Medicine mentioned how 21st century deep learning algorithms can help to overcome this. Insilico Medicine aim to extend health and longevity by using artificial intelligence for drug discovery and aging research. Polina described how the development of their new nutraceutical supplement to slow down, reduce and even reverse aging, involved using deep neural networks to screen for naturally occurring compounds that turn back the clock at the cellular level.
Pearse Keane, NIHR Clinician Scientist, Moorfields Eye Hospital, is confident that ophthalmology will be the first area of medicine to fully utilize deep learning. If a deep learning algorithm could look at an optical coherence tomography and issue a diagnosis by looking at the scan, it would completely reinvent the eye exam as we know it.
Machine learning could even enhance sight itself, as shown by Stephen Hicks, Founder and Head of Innovation of OxSight. If you can symbolize the world in a reduced form, it will be possible for visually impaired people to interact with the world more meaningfully.
Deep learning can help to determine what the most important object to pay attention to is. This is crucial for visually impaired people, as sometimes it is not the closest object. Neural networks could be changed to recognize objects – not only where they are, but also cropping to find borders of the objects.
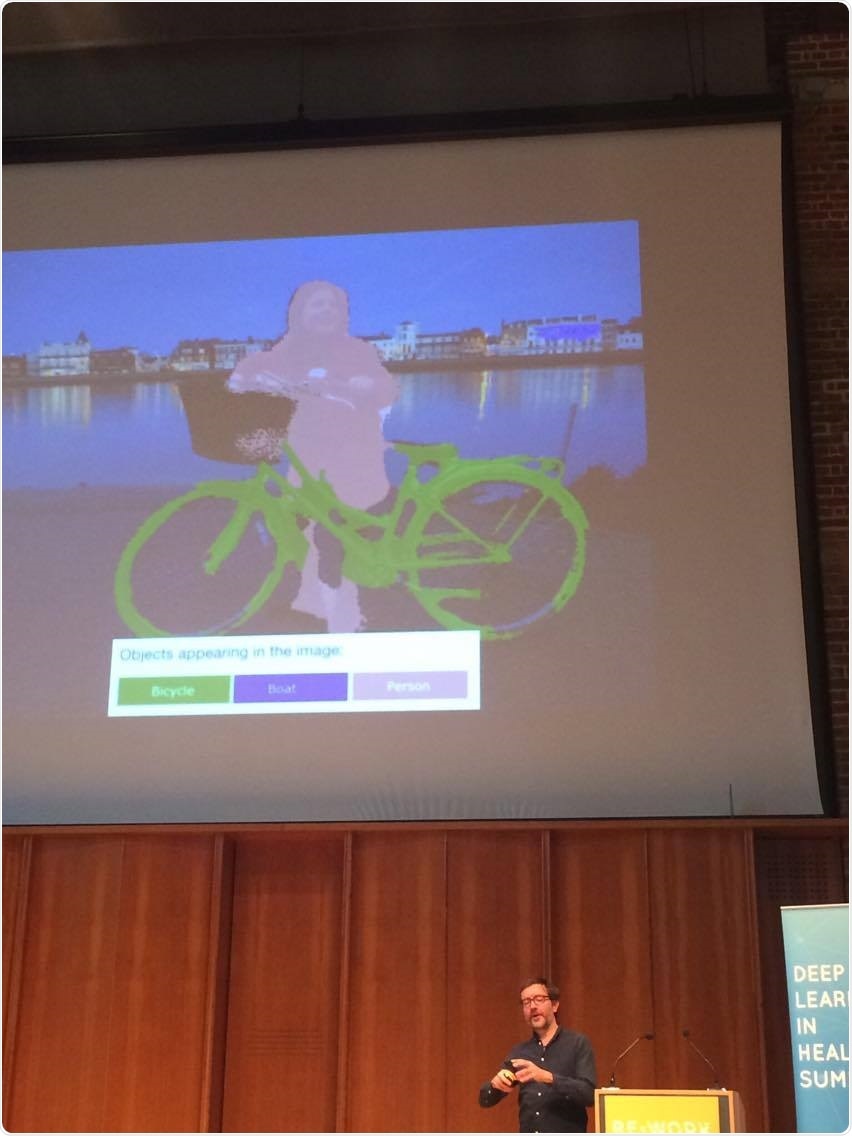
Our obsession with wearable fitness trackers was shown to have some clinical applications, as Johanna Ernst (Wearable-Technology researcher, University of Oxford) presented research from Cook et al, which showed that when looking at the recovery of patients undergoing major surgery, the number of steps tracked by wearable devices correlated with the length of time in the hospital and by looking at this they were able to tell by day 2 (post-surgery), how long these individuals would be in the hospital for.
Deep learning solutions can be incorporated into this to be able to classify more activities more accurately and identify meaningful markers. Johanna's work is focusing on predicting heart failure hospitalisation using data from wearable technologies.
Gilles Wainrib, Co-Founder of Owkin Science, emphasized the importance of transfer learning for the success deep learning as it brings the power of machine learning to small datasets. Transfer learning is a domain of artificial intelligence which focuses on the ability for a machine learning algorithm to improve learning capacities on one given dataset through the previous exposure to a different one.
This can be seen in their platform, Deepscope - a platform that can apply algorithms to analyze new images and make predictions on cancer diagnosis or chemotherapy response predictions. This platform also has the ability to share algorithms to the marketplace, meaning that by using a transfer learning technology like this, algorithms can cross-fertilize and therefore circumvent the data sharing problem.
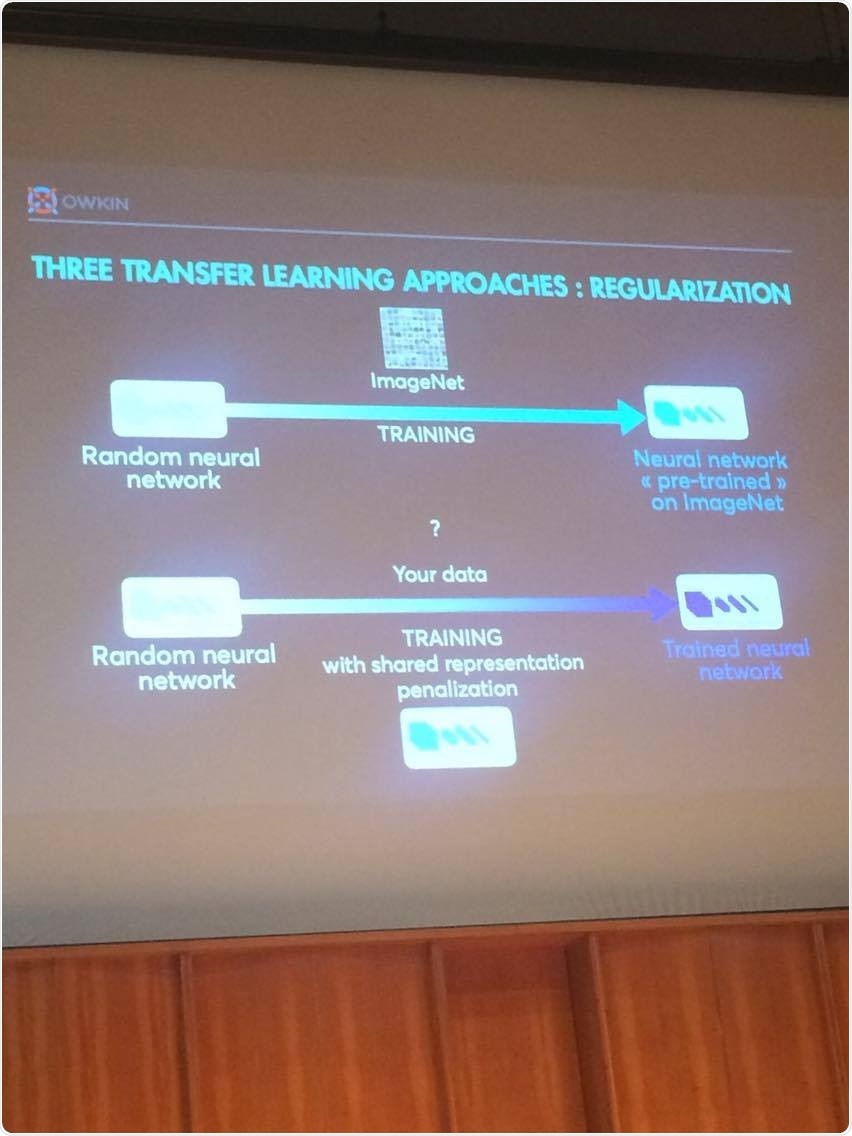
Valentin Tablan, Principal Scientist showed that neural networks also have a place in mental health. Ieso Health's online talking therapy conists of patients talking to a human therapist, via on-line messaging. The system was shown to help mental health patients get better faster compared to results for face-to-face therapy, averaged nationally from NHS Digital public data. The neural networks work presented was about building tools to support the therapists, making them more effective and efficient.
The concept of computers and algorithms replacing doctors was also covered by Daniel Nathrath, Co-Founder of Ada Digital Health. Here, a symptom checker “chat-bot” app has been created to help the public to identify what is wrong with them based on their symptoms.
Ada + Alexa = hands-free health!
The app supports doctors too as patients can share their assessment with their doctor. The technology in the app benefits from this as Ada have created a continuous feedback loop which means that the doctor’s analysis of the diagnosis means that the app gets smarter with every case that it works on as it continuously learns from the feedback.
Although deep learning in healthcare comes with its challenges, such as difficulties teaching the system to learn the right features and learning how to discriminate, detecting when the system goes wrong and difficulties going beyond human level performance, as summarized by Ben Glocker, Lecturer in Medical Image Computing at Imperial College London, it is apparent that deep learning has a massive potential to transform healthcare.
This is through improving patient quality of life, diagnosis, treatment and assessing response to treatment. Although it is very unlikely that algorithms will completely replace human doctors, it can at least relieve the pressure on doctors for basic diagnosis and administrative tasks.