Machine learning (ML) models have been increasingly used in clinical oncology for cancer diagnosis, outcome predictions, and informing oncological therapy planning. The early identification and prompt treatment of cancer, revolutionized by rapid and precise analysis of radiological and pathological images of tissues using ML algorithms, can improve the likelihood of survival and quality of care provided to cancer patients.
In a recent review published in the journal Cell, researchers at Stanford University review the application of ML in improving cancer diagnosis, treatment, and prognosis.
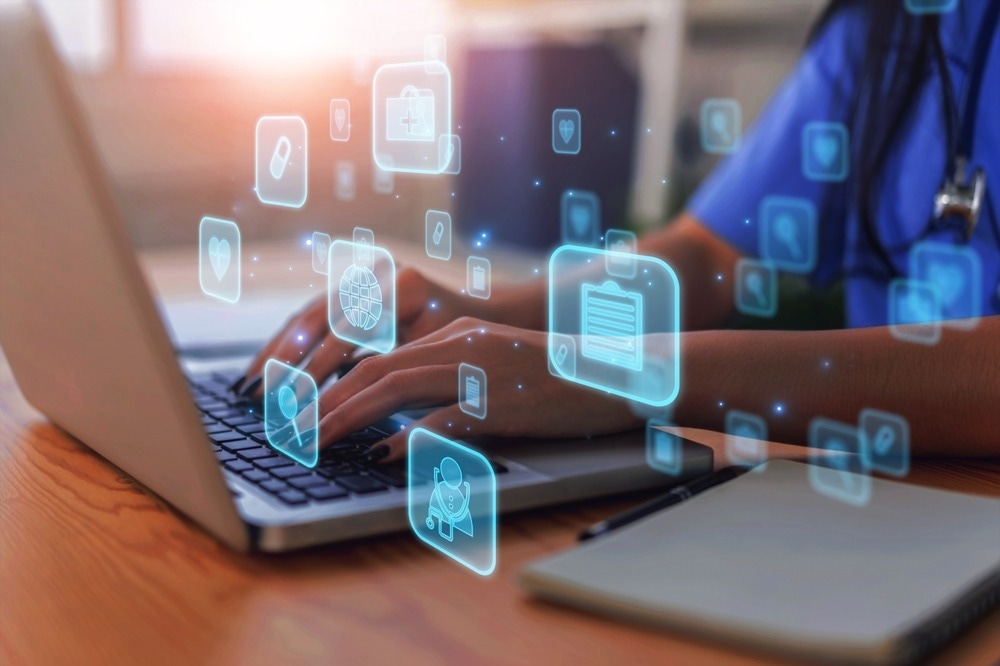
Study: From patterns to patients: Advances in clinical machine learning for cancer diagnosis, prognosis, and treatment. Image Credit: Have a nice day Photo / Shutterstock.com
Common ML models in oncology
ML models are based on supervised learning, with each data point having an associated label. Commonly used ML models include random forest models, support vector machines (SVMs), regression models, neural networks, recurrent neural network (RNN) models, convolutional neural network (CNN) models, transformers, and graph neural network (GNN) models.
Random forest models make estimations by building decision-making trees based on several binary decisions for the inputs. SVM models provide lines or multidimensional hyperplanes for tumor features by separating different data point classes from the largest probable margination between data classes. Regression models combine inputs linearly to estimate continuous labels and binary labels by linear regression and logistic regression, respectively.
Neural networks comprise several neuronal layers iteratively computing linear-type assimilations of input variables followed by non-linear functions to estimate outcomes like cancer probability. RNN models process sequential information, including genomic sequences and image series, by applying similar layers of neural networks to all objects present in the sequences and memorizing the observed objects.
CNN models apply neural patches or ‘filters’ that scan images and identify patterns. The initial layers detect low-level characteristics such as edges, whereas subsequent layers detect high-level characteristics like the morphology of tumor cells. Transformers analyze sequential information by repeated application of the attention operation for comparing the sequential to other components and updating internal sequence representations.
GNN models assess graph-structured information such as cell-to-cell interaction graphs. The models encode basic characteristics of the nodes and edges in the graphs. This information is then passed by the layers of the neural networks as they move across ML graphs for updating corresponding representations.
The representations are utilized to estimate graph labels. All general model classes have particular architecture and differ in their neural network layer size and number.
ML for and cancer diagnosis, prognosis, and treatment
For every patient, images are captured using pathological, radiological, and other imaging modalities. The high-resolution image is broken down into image tiles that span the entire image or only the region of interest (ROI) for processing by ML models. CNN models process the image tiles and generate pixel- or tile-level predictions, with heatmaps predicting sites where tumors are likely to arise.
Further, tile-level outputs are aggregated into one output using formulas or ML models like the RNN. The final estimation components, like the neural networks, use the integrated tile output for label predictions that are assessed using metrics. Labels may be obtained from various sources, such as biopsies or radiology, and could be of several types including binary labels for tumour classification and real-valued labels for tumor regression.
Radiology images are used to detect potentially malignant lesions at the time of regular screening or for symptomatic cases. If radiology images suggest cancer, biopsies are obtained and the diagnosis is confirmed by analyzing the histopathological images. Radiology and pathology images are also used for prognostic evaluation and selection of the most appropriate therapy.
Common molecular datasets, which can be obtained by single-cell transcriptomics and spatial proteomics, bulk ribonucleic acid (RNA) sequencing of tumor biopsies, and whole-genome sequencing, include circulating cell-free deoxyribonucleic acid (cfDNA), fragmentomics, epigenetic modifications, and the status of DNA methylation. These datasets are incorporated into SVMs, elastic net models, random forest classifiers, and Bayesian models for selecting the type of and predicting response to cancer therapies.
Random forest classifiers can identify tumor origin using consecutively appearing cytosine and guanine (CpG) DNA sites and micro-RNA (miRNA). Cell-type-specific gene profiles can be inferred using ML without physically isolating cells. GNNs can predict cancer outcomes from spatial proteomics of head and neck cancers.
Elastic net models can predict the response to immunotherapy from DNA fragmentomics profiles. Data considerations for ML include the signal-to-noise ratio, sparsity, dimensionality, and feature selection.
Several ML medical devices for cancer have been authorized by the United States Food and Drug Administration (FDA) and Clinical Laboratory Improvement Amendments (CLIA) for use in breast cancer mammography, gastrointestinal endoscopy, and detecting prostate cancer from magnetic resonance imaging (MRI) with SVMs and lung cancers from chest radiographs and computed tomography (CT) with CNNs. ML devices have also been used to detect ovarian cancers.
Conclusions
The current review highlights ML models used in oncology and the regular ML pipeline for image-based diagnostic, therapeutic, and prognostic estimations of cancer from molecular features of liquid and solid tissue samples.
ML predictions can stratify cancer risks, evaluate risk factors such as breast density for breast cancer, detect tumor cells, aid in treatment selection, and predict cancer outcomes by identifying cancer subtype, mutational status, tumor metastasis, microsatellite instability, patient survival, and response to radiotherapy, chemotherapy, and immunotherapy.
Journal reference:
- Swanson, K., Wu, E., Zhang, A., et al. (2023). From patterns to patients: Advances in clinical machine learning for cancer diagnosis, prognosis, and treatment. Cell. doi:10.1016/j.cell.2023.01.035