In a recent study published in the journal Nature Medicine, researchers developed and validated an Artificial Intelligence (AI) model that uses multimodal data to accurately differentiate between various dementia (significant cognitive decline) etiologies for improved early and personalized management.
Study: AI-based differential diagnosis of dementia etiologies on multimodal data. Image Credit: PopTika / Shutterstock
Background
Dementia, which affects nearly 10 million people annually, poses significant clinical and socioeconomic challenges. Precise diagnosis is critical for effective treatment, yet it is challenging due to overlapping symptoms among various types. As populations age and the demand for accurate diagnostics in drug trials grows, the need for improved tools becomes urgent. The shortage of specialists exacerbates the issue, highlighting the necessity for scalable solutions. Further research is needed to evaluate the impact of the AI model on healthcare outcomes and its integration into clinical practice.
About the study
The present study involved 51,269 participants from nine cohorts, collecting comprehensive data including demographics, medical histories, lab results, physical and neurological exams, medications, neuropsychological tests, functional assessments, and multisequence Magnetic Resonance Imaging (MRI) scans. Participants or their informants provided written informed consent, and protocols were approved by institutional ethical review boards. The cohort included individuals with normal cognition (NC) (Healthy brain function, 19,849), mild cognitive impairment (MCI) (slight cognitive decline, 9,357), and dementia (22,063).
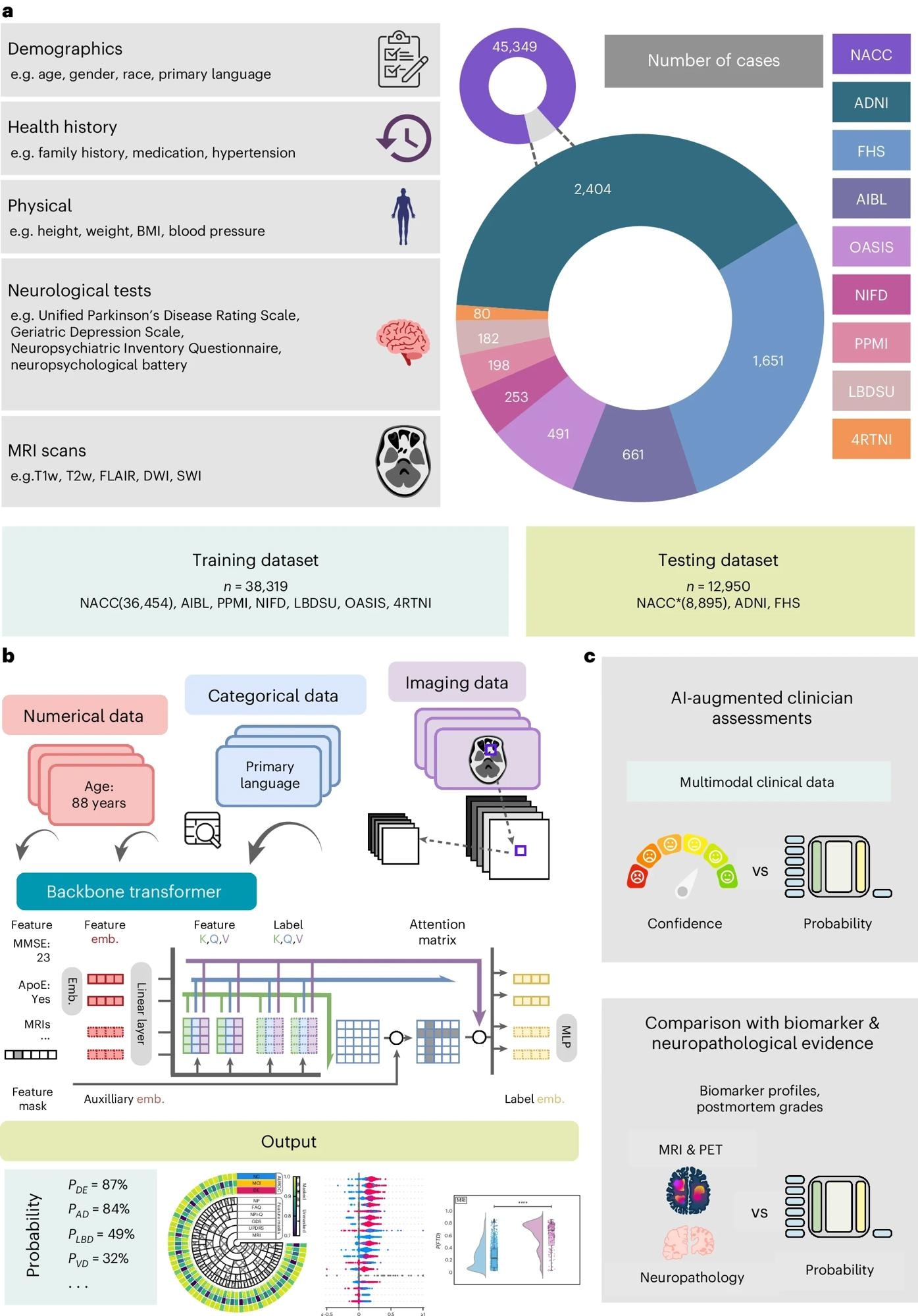
a, Our model for differential dementia diagnosis was developed using diverse data modalities, including individual-level demographics, health history, neurological testing, physical/neurological exams and multisequence MRI scans. These data sources whenever available were aggregated from nine independent cohorts: 4RTNI, ADNI, AIBL, FHS, LBDSU, NACC, NIFD, OASIS and PPMI (Tables 1 and S1). For model training, we merged data from NACC, AIBL, PPMI, NIFD, LBDSU, OASIS and 4RTNI. We used a subset of the NACC dataset for internal testing. For external validation, we utilized the ADNI and FHS cohorts. b, A transformer served as the scaffold for the model. Each feature was processed into a fixed-length vector using a modality-specific embedding (emb.) strategy and fed into the transformer as input. A linear layer was used to connect the transformer with the output prediction layer. c, A subset of the NACC testing dataset was randomly chosen to conduct a comparative analysis between neurologists' performance augmented with the AI model and their performance without AI assistance. Similarly, we carried out comparative evaluations with practicing neuroradiologists, who were provided with a randomly selected sample of confirmed dementia cases from the NACC testing cohort, to assess the impact of AI augmentation on their diagnostic performance. For both these evaluations, the model and clinicians had access to the same set of multimodal data. Finally, we assessed the model’s predictions by comparing them with biomarker profiles and pathology grades available from the NACC, ADNI and FHS cohorts.
Dementia cases were further classified into Alzheimer's disease (AD) (memory loss dementia, 17,346), Lewy body (hallucinations and motor issues) and Parkinson's disease (movement disorder with dementia) (LBD, 2,003), vascular dementia (VD) (cognitive decline from reduced brain blood flow, 2,032), prion disease (PRD) (rapid neurodegenerative disorder, 114), frontotemporal dementia (FTD) (personality and language decline, 3,076), normal pressure hydrocephalus (NPH) (fluid buildup causing dementia-like symptoms, 138), dementia due to systemic and external factors (SEF, 808), psychiatric diseases (PSY, 2,700), traumatic brain injury (TBI, 265), and other causes (ODE, 1,234).
The study utilized data from the National Alzheimer's Coordinating Center (NACC), Alzheimer's Disease Neuroimaging Initiative (ADNI), Frontotemporal Dementia (FTD) Neuroimaging Initiative (NIFD), Parkinson's Progression Marker Initiative (PPMI), Australian Imaging, Biomarker and Lifestyle Flagship Study of Ageing (AIBL), Open Access Series of Imaging Studies-3 (OASIS), 4 Repeat Tauopathy Neuroimaging Initiative (4RTNI), Lewy Body Dementia Center for Excellence at Stanford University (LBDSU), and the Framingham Heart Study (FHS). Eligibility required NC, MCI, or dementia diagnosis, with NACC data as the baseline. Data from other cohorts were standardized using the Uniform Data Set (UDS) dictionary. An innovative model training approach addressed missing features or labels, ensuring robust data utilization and maximizing sample sizes.
Study results
This study leverages multimodal data to rigorously classify dementia into thirteen diagnostic categories defined by neurologists, aligning with clinical management pathways. LBD and Parkinson's disease dementia are grouped under LBD due to similar care paths, while VD includes cases with stroke symptoms managed by stroke specialists. Psychiatric conditions like schizophrenia and depression are categorized under PSY.
The model demonstrated strong performance on test cases of NC, MCI, and dementia, achieving a microaveraged Area Under the Receiver Operating Characteristic Curve (AUROC) of 0.94 and an Area Under the Precision-Recall Curve (AUPR) of 0.90. It outperformed CatBoost on Alzheimer's Disease Neuroimaging Initiative (ADNI) and Framingham Heart Study (FHS) datasets, highlighting its superior diagnostic accuracy.
Shapley analysis identified key features influencing diagnostic decisions: cognitive status, Montreal Cognitive Assessment (MoCA) scores, and memory task performance for NC predictions; memory-related features, functional impairment, and T1-weighted MRI for MCI predictions; and functional impairment, lower Mini-Mental State Examination (MMSE) scores, and Apolipoprotein E4 (APOE4) alleles for dementia predictions.
The model demonstrated resilience to incomplete data, maintaining reliable scores even with missing features. Despite significant missing data, validation on external datasets like ADNI and FHS showed strong performance, with weighted-average AUROC and AUPR scores of 0.91 and 0.86 for ADNI and 0.68 and 0.53 for FHS, respectively.
In assessing alignment with prodromal Alzheimer's disease (AD), the model consistently attributed higher AD probabilities to MCI cases associated with AD, reinforcing its utility in early disease detection. Comparison with Clinical Dementia Ratings (CDR) across the NACC, ADNI, and FHS datasets strongly correlated with CDR scores, highlighting the model's sensitivity to incremental clinical dementia assessments.
The model exhibited strong diagnostic ability across ten distinct dementia etiologies, with microaveraged AUROC and AUPR values of 0.96 and 0.70, respectively. Although variability in AUPR scores indicated challenges in identifying less prevalent or complex dementias, the model performed robustly across demographic subgroups.
Aligning model-predicted probabilities with AD, FTD, and LBD biomarkers, the model showed strong differentiation between biomarker-negative and positive groups, validating its effectiveness in capturing dementia pathophysiology. Postmortem data validation further supported the model's capability to align probability scores with neuropathological markers.
AI-augmented clinician assessments showed significant improvements in diagnostic performance, with increased AUROC and AUPR scores across all categories, demonstrating the model's potential to enhance clinical dementia diagnosis.
Conclusions
The study introduces an AI model for differential dementia diagnosis using multimodal data. Unlike previous models, it distinguishes between various dementia etiologies, such as AD, VD, and LBD, which are crucial for personalized treatment strategies. Validated across diverse cohorts, the model's predictions were corroborated with biomarker and postmortem data. Combining model predictions with neurologist assessments outperformed neurologist-only evaluations, highlighting its potential to enhance diagnostic accuracy. The model addresses mixed dementias by providing probability scores for each etiology, improving clinical decision-making.